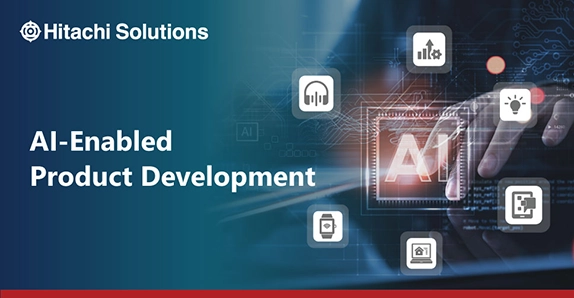
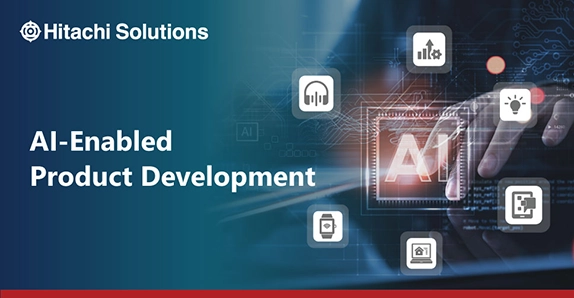
Predicting the right product to build, investing in prototypes, and testing those assumptions can be a long and expensive process. But does it have to be? It’s a new world for product development with generative AI. Generative AI isn’t only a technology— it’s a tool that can synthesize customer feedback, identify and design new products, and fine-tune existing ones.
As generative AI technologies become more accessible and cost effective, they are destined to reshape how companies conceive, design, test, and launch products. How can they do that? Let’s take a look at this emerging world of product development and design and how AI can be used speed up the development cycle and help teams create products that better reflect customer needs and wants.
Making Real-time Feedback Actionable
The potential impact of being able to convert product feedback into actionable data points is enormous. Traditionally, many companies have relied on focus groups and anecdotal feedback, but those means aren’t always accurate representations of customer sentiment, and can leave product teams vulnerable to creating products that don’t actually serve customers.
Most enterprise information is unstructured, in the form of documents, emails, social media feedback, and product comments. Generative AI can analyze that feedback along with internal company data to figure out which features customers are talking about the most, or what response customers have when it comes to particular product features, for example.
If you’re not analyzing this unstructured data, your company may be missing out on more than half of the data that can help inform product direction.
The LEGO Group is one company that is using market insights tools powered by AI to analyze emerging trends and predict product growth based on publicly available data from the Internet, such as likes or clicks on trending videos or memes. The company then shares this information with product developers to increase their understanding of consumer and shopper trends. Product teams are able to more quickly identify whether a product is meeting market needs, and even features that should be added or removed from a product.
When using AI to complete a lot of the front-end homework, it’s clear companies will have more robust results. Research has shown that companies that shape product development using data have significantly higher rates of new product success compared to those that do not.
Accelerating the Ideation Process
Physical prototyping can be time consuming and expensive. Being able to digitally verify designs can dramatically reduce the lead time for product development. AI can help speed up the product development process with rapid prototyping and algorithms can optimize design plans quickly by running thousands of simulations a minute, so teams can quickly identify the most promising option and iterate in real-time.
For example, AI can take a traditional problem – like creating drawings for a new concept car – and predict how an artist would draw the car, given specific instructions. Data-driven prediction is at the heart of making decisions under uncertainty, and that’s a major part of creating any new product, and hence the transformative role for AI.
In another example, product developers could use generative AI to identify features that are in one product but not another by feeding the product documentation for their product and a competitor’s product into a generative AI tool. Then they can prompt the tool, asking it to determine what features are in one product and not the other, getting insight within seconds. We all know product research groups have been manually doing this for ages, but imagine how much time they could save with these tools.
According to a report by IDP Innovation, AI is already cutting development times by 50 percent for leading early-adopter firms.
Institute of Product Development
Testing Real-world Scenarios
Product testing should be an iterative, continuous process. Smart companies constantly evaluate and test products, adapt, change and repeat. Generative AI tools drastically cut down the friction-in-iteration identifying defects and errors, analyzing test results, and generating reports, resulting in improved product quality.
AI-powered simulations can be used to test how a product will perform under different conditions, allowing for early identification of potential issues and optimization of designs. It can also help engineers identify and eliminate risks related to safety and human factors, reducing the likelihood of product recalls and improving user satisfaction.
For example, Facebook employs AI-powered testing tools to automatically generate and execute thousands of test cases, identifying issues in their code before it reaches users. This approach reduces the time and resources needed to test products while increasing their quality and reliability.
It’s always important to remember that while technology is great for building prototypes and automating testing, the human element— feedback from potential users and customers— is irreplaceable.
Honing in on Product-to-Market Fit
Product-to-market fit (PMF) means creating a profitable product that keeps individual users coming back. Product development requires deep analysis of user feedback that can help make your product-market fit predictions more accurate. The conversation around how to achieve product-market fit is coming up more frequently and much sooner than ever before across industries, due to increasing competition and changing consumer behavior.
To be worth investing in, a product needs to solve a customer need quicker, easier, and better than competitors. To do so, companies need to understand which features are most valuable to their customers (and why). It’s almost impossible to scale product-led growth without that information. Using generative AI to synthesize product data from customers can provide a clear roadmap of where to take a product next.
At Hitachi Solutions, we use Generative AI to assist our own product teams. We consolidate customer surveys, user traces, and emails about product issues into one location, then interrogate the data with AI models to help us find patterns and common areas of interest. After analyzing these results, we use the information to reposition our feature roadmap if needed.
Improving Product Personalization
Because all brands are now using personalization to engage customers, it’s critical to consider how AI can bolster efforts to reach individual consumers. According to Salsify research, 70% of U.S. shoppers are more likely to buy an item if there are personally relevant images, text, and reviews for it.
Stitch Fix, an online personalized styling service, is a prime example of how AI revolutionizes product development. The company uses AI algorithms and human stylists to curate personalized clothing selections for their customers. By analyzing customer data, preferences, and feedback, Stitch Fix’s AI algorithms can identify trends, predict customer needs, and recommend items most likely to resonate with individual clients. Recently, the company began experimenting with DALL-E 2 to create visualizations of clothing based on requested customer preferences for color, fabric, and style.
Opening New Avenues for Creativity
Product development should be a collaborative process, with the goal of getting different perspectives that will spur innovation. Generative AI can supplement that process, providing valuable analysis that can evaluate the quality and applicability of a product team’s ideas, and even generate better ones.
I can’t recommend replacing the human element; the truth is humans are already incredibly brilliant and creative in our own right. However, AI can provide inspiration, spark ideas and spur creativity during the everyday processes in product development. Think of the potential using it in areas like design and art. Through refined modeling, generative AI can get a deeper understanding of the thinking behind the creative process, enabling more individuals to bring ideas to life.
Challenges and Caveats
If you’re using generative AI to assist with product design or development, it’s important to remember that successful design is closely tied to the context in which the product will be used, and AI outputs do not recognize nuances that are critical to solving the customers’ problems. Instead, AI may offer generalized advice or ideas that overlook key aspects of an issue.
That’s why the importance of human oversight can’t be overstated. You can’t always maintain control of content produced using generative AI. These AI models can even produce undesirable and unexpected outputs that need to be verified, corrected manually, or thrown away entirely. The best way to ensure that your AI models are properly trained is to have a generate-and-test process that ensures employees communicate errors and uncertainties in the outputs if they appear to be suspect.
How Hitachi Solutions Stands Out
As we see the technology evolve, the use cases for using generative AI in product engineering and development will only expand further. The gulf of efficiency is only going to grow larger between the teams who use AI to accomplish tasks like product development and the teams that do not. Take it from us: opening up your work processes to new AI tools can be a daunting prospect, but the results can be well worth the effort.
In the early stages of your company’s data maturity journey, you’ll want to remain focused on your highest priorities, and how your business can get the most out of AI. If you’re interested in learning more about how you can use AI technology, contact us today! We’re 100 percent Microsoft-focused, and have teams dedicated to advancing AI solutions across all industries.