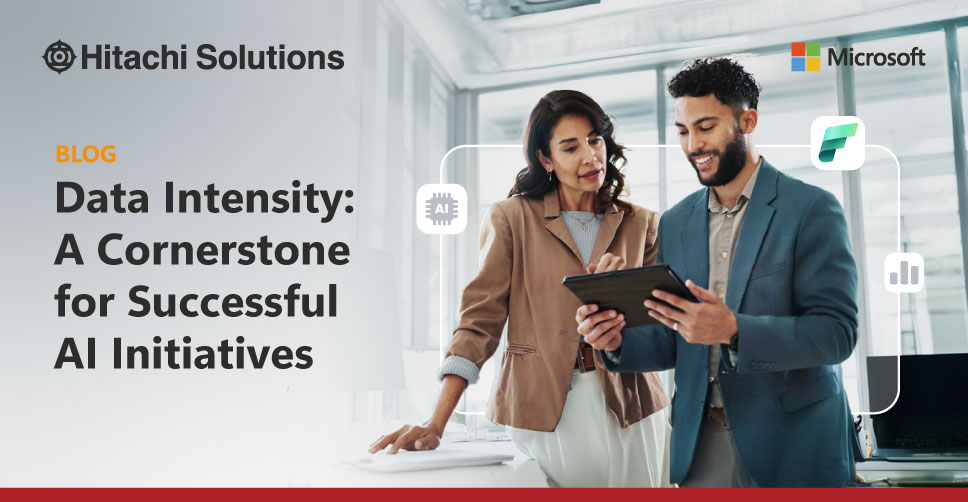
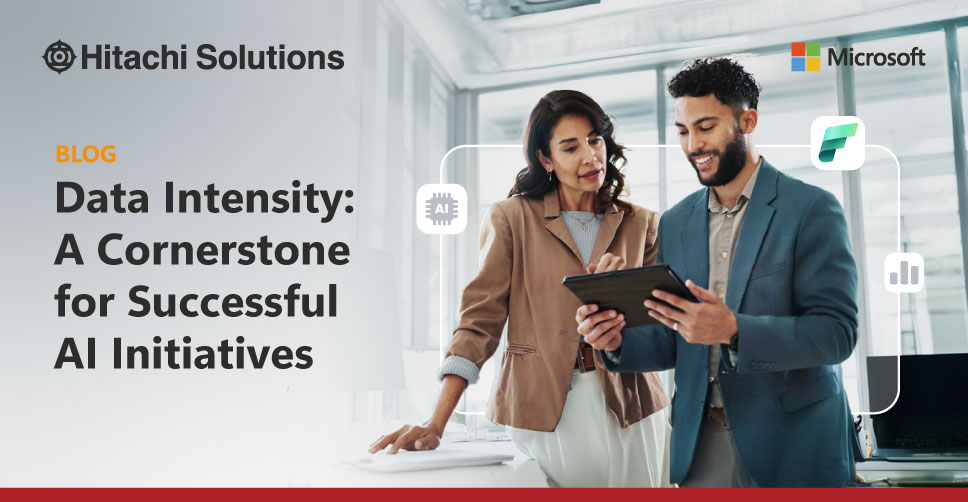
The best AI tools use the best data, and the best decisions are made when you have the whole picture.
When it comes to analytics and artificial intelligence in field service, this is absolutely true, and one principle stands out above the rest: data intensity. The combination of data volume and quality is vital to building robust AI models and making accurate business decisions.
In a recent webinar, I was joined by two fantastic guests – Dhruv Goel, Microsoft’s Principal Group Product Manager for Dynamics 365 Field Service, and
Luis Masieri, Principal Group Product Manager for Microsoft – to discuss the critical steps that field service leaders need to take to make their AI vision a reality.
Watch the clip below to hear us discuss the importance of data intensity, and break down some of the myths around data volume, quality, and what it takes to get to predictive maintenance and artificial intelligence.
Let’s break down some of the topics discussed in this clip.
Understanding Data Intensity: Data intensity refers to the dual necessity of having a large amount of data (volume) and ensuring that this data is of high quality.
The Role of Data Volume in AI: The volume of data is crucial because it allows AI models to learn from a wide range of scenarios and patterns, leading to more accurate and reliable outputs. For example, a sales prediction model trained on data from thousands of transactions will outperform one trained on just a few hundred.
The Importance of Quality Data: However, volume alone isn’t enough. The data must also be accurate, relevant, and free from biases. Poor quality data can lead to incorrect predictions and business decisions. Ensuring data quality involves processes like data cleansing, validation, and continuous monitoring.
Combining Volume and Quality for Optimal AI: Achieving both volume and quality is essential for leveraging AI effectively. Here are some steps to ensure high data intensity:
- Data Collection: Implement robust data collection methods to gather comprehensive datasets.
- Data Cleaning: Regularly clean and validate data to remove errors and inconsistencies.
- Data Integration: Use platforms like Microsoft Fabric to integrate various data sources into a unified framework.
- Continuous Monitoring: Keep an eye on data quality and update the dataset regularly to maintain its relevance.
The success of AI initiatives hinges on data intensity. By investing in both data volume and quality, businesses can unlock the full potential of AI to drive innovation and competitive advantage. To delve deeper into this, and to hear how you can turn AI potential into actual value in field service, I invite you to watch our full webinar.