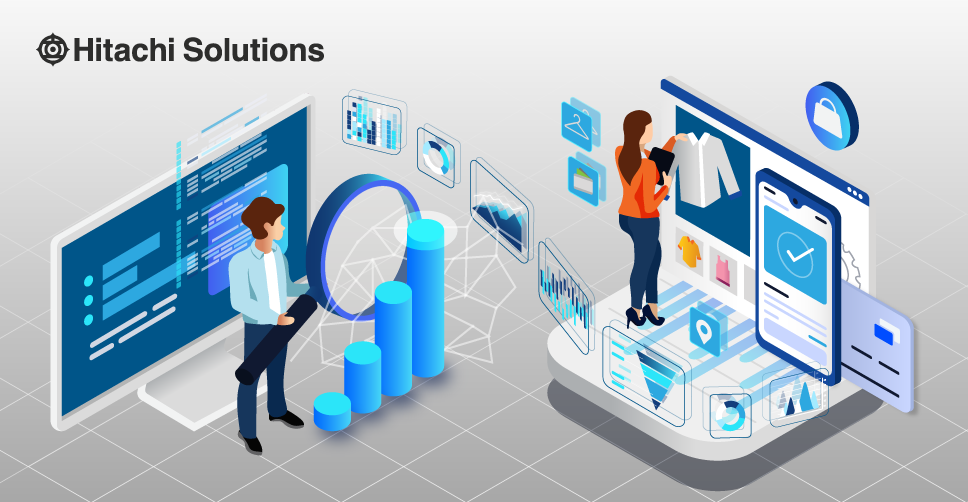
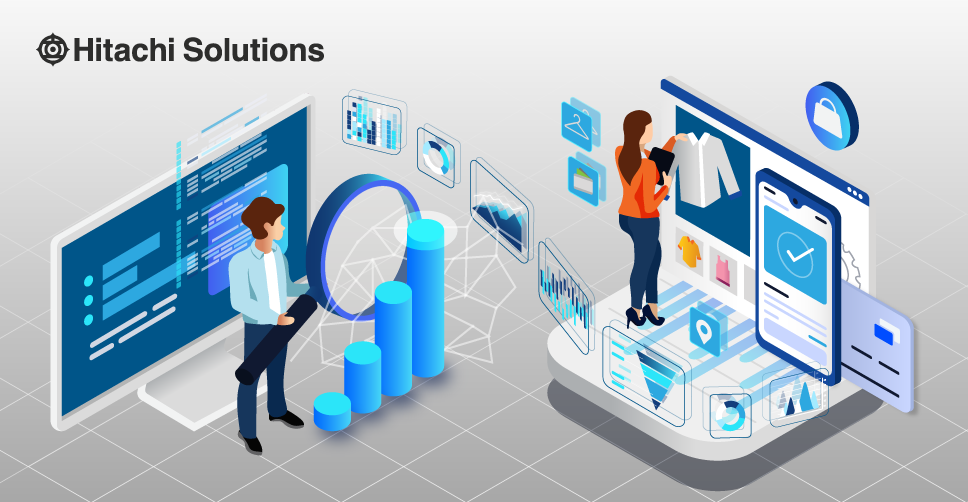
Retail and CPG App-in-a-Day
Dive into Power Apps to stay agile, responsive, and profitable in our Microsoft Workshop
Watch the On-Demand WebinarOver the last few years, across all industries, the amount of data used to make future decisions has exploded. Not only does the tremendous volume of data threaten to overflow traditional data warehouses; it moves so fast that – without the capability of near real-time processing – the next decision is made on stale data, becoming nothing more than a guess.
Retail organizations feel this pain acutely as they need to navigate an overwhelming number of data points, all to serve customers who have come to expect a Netflix-level experience of personalization and curation at every retail interaction. Fortunately, predictive analytics can allow retail decision-makers to be proactive, rather than reactive, in determining how to maximize sales opportunities and revenue.
What Is Retail Predictive Analytics?
Predictive analytics is the scientific discipline using statistical modeling, such as regression, classification, and clustering, that consumes existing data to identify relationships in data and anticipate trends to make predictions on future business.
The most common example, especially when it comes to retail customers, is when online services offer recommendations based on past purchases or viewing history – a type of automatic cross-selling that drives customers to the content they’re more likely to be interested in. Another common example is the ability to forecast an increase in customer demand, which in turn can enable retailers to prepare for possible product shortages.
Creating retail predictive analytic tools is different from traditional business intelligence, which usually only mines historical data for trends and then describes what happened in the past. Instead, for predictive analysis, data is pulled from a host of different sources, including:
- Mobile applications and web portals
- Customer reward programs
- Point-of-sale systems
- The retail supply chain
- In-store sensors and cameras
- Social media platforms
- Demographic indicators
- Economic indicators
- Weather models
From all this available data, predictive models can be developed that utilize AI-powered data analytics to forecast the most likely outcomes. These retail data analytics enrich insights into how to engage current customers, attract new business, enable market expansion, and future-proof business in a cost-effective manner.
7 Benefits to Utilizing Predictive Analytics in Retail
The major predictive operating opportunities for retailers include:
- The creation of more targeted marketing campaigns.
Taking all the available data on customers, including both internal transactions and external comments and demographics, allows retailers to identify the best leads at the best time, as well as which messages or offers may be more attractive to certain types of customers. - An increase in customer engagement and overall satisfaction.
Analytics makes it easier to understand what lies underneath net promoter scores by finding actionable insights across thousands of customer reviews. - Pricing products more strategically.
Dynamic price optimization is possible thanks to regularly updated supply and distribution data in coordination with demand forecasting. - Designing one-of-a-kind customer experiences.
Ensure that all sales interactions are hyper-personalized both in brick-and-mortar stores and virtual e-commerce platforms, curating your customers’ experience so it can be truly seamless. - Gaining competitive intelligence for informed decision-making.
Retail analytics provides you with better leverage of external data sets that define the competitive boundaries of your organization, allowing you to proactively identify emerging changes, opportunities, or threats. - Optimizing the omnichannel customer journey.
Insights into how customers think and what motivates their purchasing decisions allow you to understand how, when, and what are the best channels to contact sales leads – improving conversion efficiency and boosting revenue. - Efficient Operating Cost: Lakehouses are cost-efficient as all data is stored in cheap object storage like Azure Gen2 storage accounts. Additionally, all data remains under the firm’s ownership, there are no egress fees to get at your own data.
8 Ways to Use Retail Predictive Analytics
There are a few important considerations before making use of predictive analytics. In every case, you will need to:
- Have good data, and lots of it — All those possible data points are excellent sources of input, but you’ll want to make sure the data is up-to-date and relevant for what you need to forecast.
- Employ the right predictive modeling technique — Cluster models? Outlier models? Regression models? Understanding which models work best for identifying patterns in data sets is key for getting the results you want.
- Account for some ambiguity — If there’s one thing to be learned from the start of the 2020s, it’s that there are always variables that will disrupt expected patterns. While it’s impossible to account for every severe weather event or global conflict, it’s important to leave some leeway in predictions.
- Have actionable insights —Insights for their own sake aren’t especially useful. Your results will need to be presented in a way that allows your team to make informed decisions that will positively affect your customers (and your bottom line).
Once these fundamentals are settled, then you can move towards employing specific retail predictive analytics. For retailers, this could touch everything from product ordering to customer marketing. Here are eight retail analytics that can provide the biggest impact for your business:
Forecasting product demand – One of the most straightforward uses of predictive analytics is in the examination of previous product sales history and demand across all available channels, which will then deliver real-time sales reports to field offices through PowerBI dashboards. This can inform decisions about which products to stock, which to feature, and which may need to be discounted. The ultimate benefit is that there are plenty of products available where and when they’re needed most – and not gathering dust where they’re not.
Forecasting market trends – Sometimes future demand isn’t based on internal history but is largely dependent upon external factors. Predictive analytics can be employed here as well, consolidating market reports while also incorporating other relevant variables such as local impacts from weather or economic changes. This can help to determine if there’s going to be a higher (or lower) demand for certain products.
Conducting market basket analysis – This is a determination of which products are purchased together, as well as which products are more popular during certain promotions or holidays. Having solid point-of-sale data here can allow retailers to better synergize how they promote and sell products. Effective use of market basket analysis enables the ability to forecast shelf level sales, improve promotional distribution, and take advantage of cross-selling opportunities.
Price optimization – It’s a delicate balancing act to keep the price of products at a level where retailers can turn a profit while maximizing sales. If prices are too high, it may turn away potential buyers. Too low, and there goes the bottom line. Today’s hyper-competitive markets mean that retailers can’t afford to maintain static prices, so price optimization requires retailers to be able to adjust pricing dynamically. Predictive analytics and scenario modeling can help determine how a new price will perform in a specific set of circumstances, which takes the guesswork out of what prices to set and when to set them.
Fulfillment optimization – Getting a product to a customer as quickly as possible is essential for raising customer satisfaction levels and ensuring repeat customers. A smart fulfillment strategy is also essential for reducing costs and making the most of valuable warehouse space. Using retail analytics allows for the combination of real-time inventory and distribution status to make the most effective use of available resources, including allowing stores to act as fulfillment centers.
Inventory and supply chain management – Ensure that products are available at the right location, at the right time, and in the right quantity with predictive analytics related to supply chain management. Attempting to react to disruptions in the supply chain or adjusting to account for product shortages is just mitigating harm rather than preventing it. Predicting likely outcomes or adjusting for future scenarios allows for proactive decisions, which can help retailers avoid shortages, maximize the value of products with expiration dates, and reduce promotional forecast errors – all of which can save millions in wasted products.
Granular customer segmentation – Personalization is more important than ever for successful marketing, and that starts with separating customers into discrete groups based on shared characteristics. While it’s possible to personalize material based on a customer’s buying habits, customer segmentation drives better purchasing predictions based on the behaviors of the available population. Using sales data, campaigns, and promotions systems, retail analytics can derive features that capture the behavior of various households, making it possible to effectively market to them before they ever step foot in a store or visit a website.
Customer journey orchestration – With today’s active customers you can’t afford to “set and forget” the customer journey; customers need to be engaged in real-time. If a customer opens a mobile application for the first time, your system could proactively point out features that could be useful. Sentiment analysis on a customer’s outreach to customer service can identify the type of issue they’re having and suggest helpful resources. Rather than trying to adjust the journey after problems occur or opportunities are lost, retail predictive analytics attempt to identify improvement opportunities while a customer is active in the sales process.
Retail Predictive Analytics for Mid-Market Companies
Implementing retail predictive analytics is often easier for enterprise-scale companies, which have the benefit of extensive internal resources, such as a larger budget, in-house data science expertise, an established infrastructure, or other advantages. However, mass-market companies aren’t the only enterprises that can benefit from retail predictive analytics; mid-market companies can (and should!) take advantage of the opportunities as well.
Investing in retail predictive analytics can feel like too big of a gamble, but not taking advantage of all the available retail data is the bigger loss for mid-market companies. There are a few helpful steps a company can take when getting started with retail predictive analytics to ensure that they’re on the right track.
- Do some research – What are other companies, competitors, and collaborators alike doing with their Big Data? Are there specific tools or solutions that are common within your market? Do you have the resources you need to get started? These are the most important questions to review first to define your next steps.
- Define the company goals – Which of the possible uses of predictive analytics is most important for your company? Is price optimization the highest priority, or is it more pressing to have more insights into the inventory and supply chain? Understanding what needs to be done first will determine what data should be prioritized.
- Determine your needs – Once you understand the insights that you’re looking for, you’ll have to account for the data points that will support it. Will you need (or can you afford) RFID tags for in-store products? What kind of data aren’t you collecting that you’ll need to account for? What kind of analytical model(s) will you need to utilize? This leads to the last recommendation…
- Find the right partner – Without the extensive resources of a large-scale enterprise to rely upon, your investments can go a lot further when you employ the right consultant or partner. If you have your own IT department, a partner can guide your team towards the right analytical models to employ and the best technology stack to support them. The right solution not only will provide the insights you’re looking for, but it should also be easy and intuitive to use.
Partner with Hitachi Solutions
Hitachi Solutions works with both mid-market and mass-market firms to jump-start their predictive analytics journey. We’ll assist you in identifying potential use cases where there is plenty of data that can be used to improve customer experience, increase revenue, or improve operational efficiency.
We can assist you with a low-cost Advanced Analytics DataLab to get your basic resources set up and analyze real data within a few weeks. By integrating the Azure Databricks unified analytics platform, we can assist you in taking the next step towards near real-time predictive insights in a cost-effective manner.