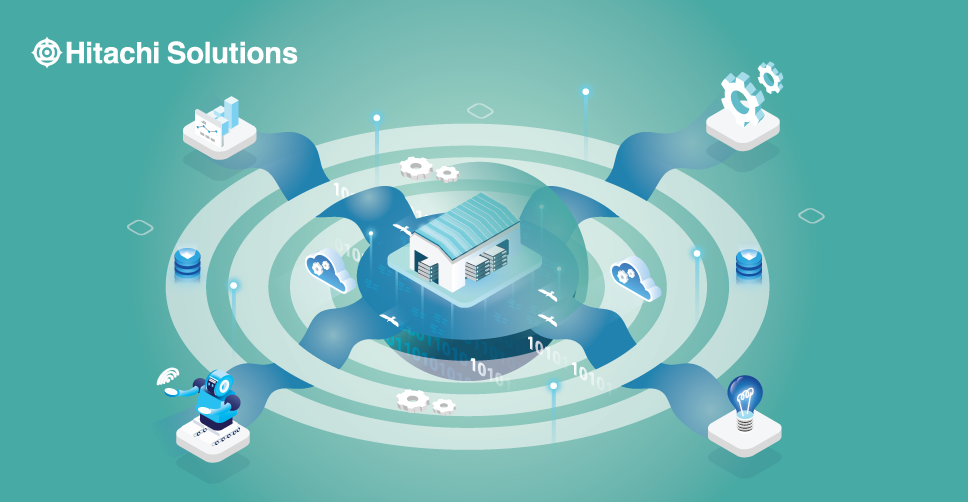
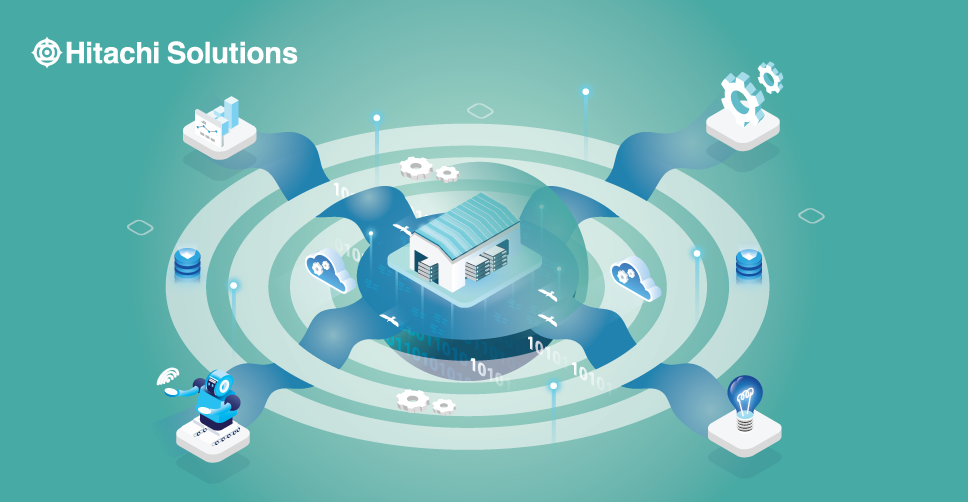
How to Approach Building a Modern Data Platform Now
We look at how to approach data platform modernization in a focused, flexible way in any type of environment — on-premises, IaaS, hybrid, or SaaS.
Read the BlogThis is the second article in a blog series on modern data architectures (read the first article here!). Today, we will explore the concepts of data fabric and data mesh and how they assist in defining an architectural framework that supports the expansion of data and analytics capabilities. If your organization is looking to maximize its use of data or seeking to modernize, this blog post is for you. At this crossroads, it’s worthwhile to consider the modern concepts discussed here to improve your data maturity.
Managing data can be challenging. The exponential growth of data, tools, and architecture left many companies finding that traditional, centralized data architectures need to be more flexible to keep up with the pace of change. In this blog post, we will dive into Data Mesh and Data Fabric, their benefits, and how they can help organizations address the bottlenecks introduced by monolithic architectures.
Here’s a simple diagram illustrating the traditional approach to data & analytics:
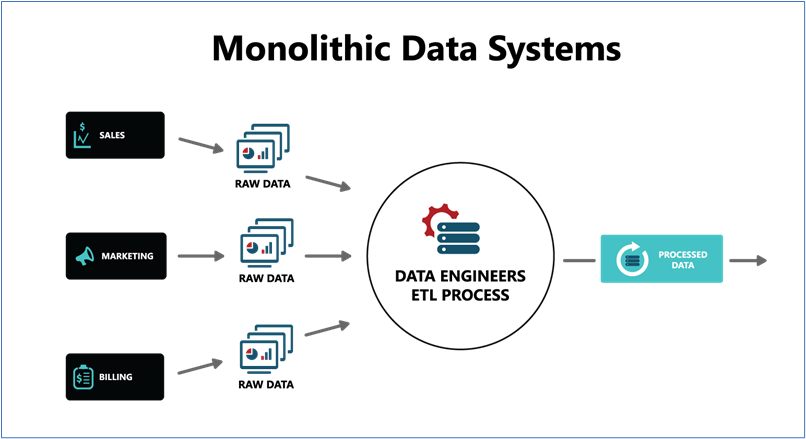
When viewing this diagram in the context of an IT-controlled data warehouse, it’s easy to see that it’s all about universal management over security, quality, access, and more. However, as adoption increases, IT becomes a bottleneck that’s overwhelmed with requests demanding more data of increasing complexity — creating consternation between those producing data and those consuming it. Simply, monolithic data systems are not optimized to scale with the ever-increasing appetite for insights. As organizations generate and collect all this data from internal and external sources, they need a way to manage it in a way that’s flexible to meet demands and scalable to grow in a controlled fashion.
Thus, new design paradigms have evolved to address the growing demand for more efficient and effective management of data. Putting this model into a distributed architecture is referred to as a data fabric. The discussion on how to handle distributed data has evolved into data mesh, with the principles of treating decentralized data as a product, with autonomy and ownership delegated to the organization’s domains.
Data mesh and data fabric are being used by organizations to improve their data management and to better meet the demands of the digital economy. They are seen as important approaches for managing data in an increasingly complex and decentralized data landscape.
Data Fabric
A data fabric design is a centralized approach to distributed data management, where a single entity acts as the center of the data universe and coordinates the flow of data between various systems, regardless of format or location. The aim is to provide a unified and seamless way to access and manage data across different systems, applications, and devices. It’s also described as a concept designed to modernize existing investments like databases, data warehouses, data lakes, data catalogs, and data integration platforms.
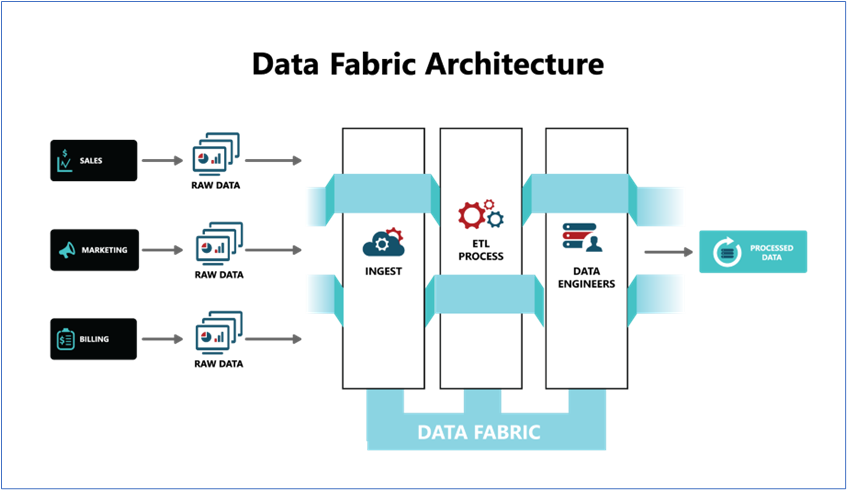
Think of it as a platform ecosystem that orchestrates disparate data sources intelligently and securely in a self-service manner. Data fabric design doesn’t require you to rip out and replace existing systems. Existing data stores and applications participate by providing metadata to the data fabric. Analytics are then applied to define metadata relationships and to find associations amongst the data — and not just data you brought together deliberately. It dramatically changes the economics of data management and starts to build insights autonomously.
Data Mesh
Data mesh is an example of a data management framework that uses a decentralized approach to share, access, and manage analytical data within or across an organization. A data mesh aims to align data products with the organizational domains such as the departments that produce the data. This approach assumes that these domains are more likely to derive value from their data. Additionally, the owning domain is likely to be the subject matter expert, enabling it to manage the complexity and challenges at the source, such as dealing with business semantics, schema changes, downtime, upgrades, and backward compatibility.
As illustrated below, each domain, such as sales, owns and manages its data and tools with the help of sales-owned data practitioners. The domain team applies product thinking to make the data discoverable, addressable, trustworthy, self-describing, and secure. This ensures effective data management by the people who understand it best and meets the organization’s needs.
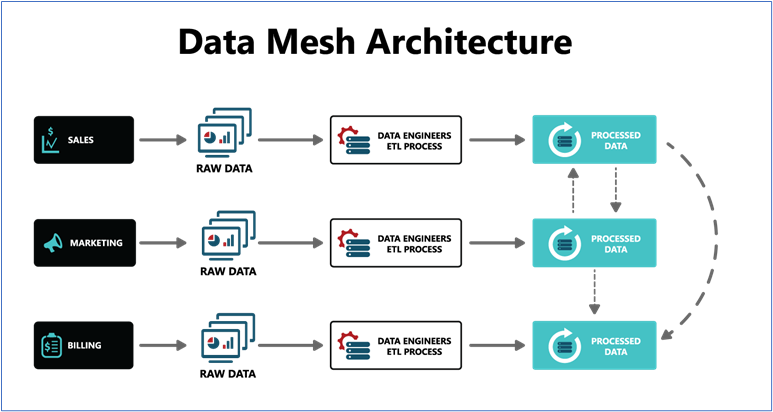
Data mesh aims to create a more scalable, flexible, and secure data architecture by allowing different teams to own and manage their data independently. Data meshes provide a solution to the shortcomings of centralized data warehouses by allowing greater autonomy and flexibility for data owners, facilitating greater data experimentation and innovation while lessening the burden on data teams to field the needs of every data consumer through a single pipeline.
How to make sense of it all
The focus of data fabric and data mesh is not on a particular product, but rather on the principles and practices that guide data management and governance within an organization. The objective is to provide a clear and consistent approach to data management, regardless of the technologies used to implement it.
Data mesh and decentralized architectures can be an effective way to implement enterprise data platforms, but it may not be the best solution for all organizations. Decentralized architectures require autonomous teams that can work independently, and often work best in large, complex organizations that need to scale analytics adoption beyond a single platform.
For all others, is it better to stick with a traditional centralized management architecture? Here’s a simple breakdown of the pros and cons of each:
Centralized Data Management (Data Fabric) | |
---|---|
PROS | |
Easy to manage and control | With all data stored in a single location, it is easier for administrators to manage and control access to the data. |
Scalability | Centralized systems are easily scalable as additional resources can be added to the central location. |
Improved security | Centralized systems can provide increased security as access to sensitive data can be restricted and monitored. |
CONS | |
Inflexibility | Centralized systems are tightly coupled with technology, making them more inflexible and unable to handle diverse user requirements. |
Lack of efficiency | Centralized systems may limit the ability of different departments to access the data they need when they need it. |
Limited data sharing | Centralized systems may limit the ability to share data with external partners, reducing opportunities for collaboration. |
Decentralized Data Management (Data Mesh) | |
---|---|
PROS | |
Flexibility | A decentralized systems approach to compartmentalization reduces risks associated with changes in budgets, teams, leadership, and technology. |
Improved accessibility | Decentralized systems allow for more flexible access to data without concerns relative to proximity. |
Increased innovation | Decentralized systems can foster agile innovation as different departments can experiment with new approaches and technologies. |
CONS | |
Complexity | Decentralized systems can be complex to set up and manage, requiring specialized knowledge. |
Cost uncertainty | Decentralized systems require more resources, potentially making them more expensive to implement and maintain. |
Data consistency | Maintaining data consistency across multiple locations can be challenging, and errors may arise if not properly managed. |
In general, companies with microservices-based applications, demanding and complex data infrastructure requirements, high data volumes, numerous data sources and domains, and large team sizes would benefit more from data mesh. For some organizations, it may be too much. On the other hand, a decentralized data architecture can improve efficiency by allowing for better sharing of data and resources across different departments, which may lead to reduced staff requirements in some cases.
There’s no right or wrong way
Ultimately, the choice between a data fabric and a data mesh — or a hybrid approach — will depend on the organization’s goals and priorities, as well as the specific challenges they face in managing and using data effectively.
A data management architecture governs how organizations collect, store, secure, arrange, integrate, and use data. A good data management architecture provides clarity about every aspect of data and how enterprises can get the best out of their data for business growth and profitability. Conversely, a bad data management architecture leads to inconsistent data, data silos, and quality problems that make data untrustworthy and can affect an organization’s ability to run analytics.
Depending upon where a company is in its data journey, tradeoffs will most likely be made. Some organizations prefer a high degree of autonomy, while others prefer quality and control. Some organizations have a relatively simple structure, while others are large and complex. Creating the perfect data architecture isn’t as easy; there’s no right or wrong.
We can help
Here at Hitachi Solutions, we’ve built an entire business unit dedicated to helping organizations derive valuable insights from big data. From our advisory services to a dedicated data science team, we offer a vast library of resources and enterprise analytics expertise.
Hitachi Solutions’ workshop-based assessment will introduce your organization to the cloud-based capabilities you can use to capitalize on the true business value of data. If you are considering modernizing your legacy data platform, our data solutions experts will work with you to identify your current data estate, define the right modern state, and determine the best path forward.
Customer maturity along the data spectrum is indeed a journey, and building and refining the right architecture over time is a key component. Stay tuned for the next installment of this blog series! If you have any questions about this post or Hitachi Solutions’ data offerings, please contact our team. We are happy to help.