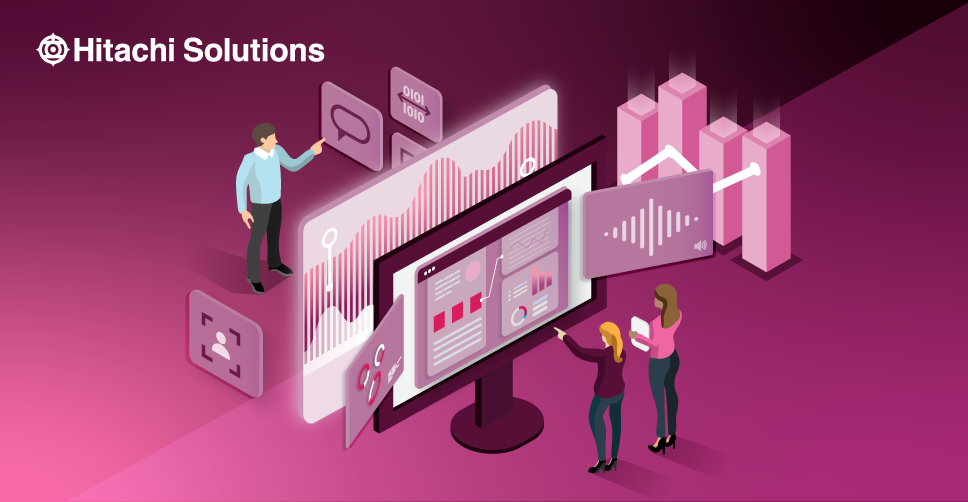
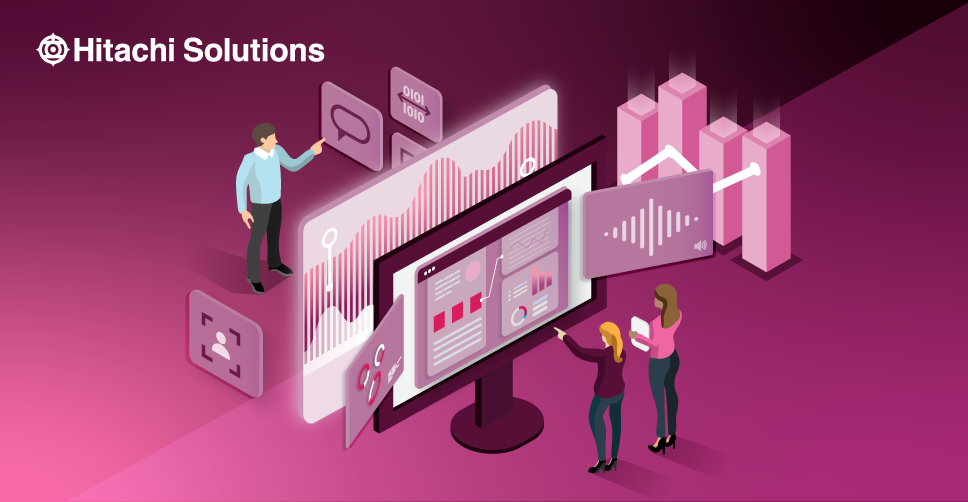
Data Science On-Demand
Practically explore your data science opportunities
Download the OfferData, data, data — it’s all we hear about. We hear about how much is created, how it’s critically important, and that businesses are leveraging it to reach their goals and increase their revenues. But how? Yes, much of it is technology based, but if there is an astronomical amount of data being generated every day, how are businesses supposed to keep up?
Well, with data science and artificial intelligence.
First, let’s start with data science.
Data science uses a combination of various tools, algorithms, formulas, and machine learning principles to draw hidden patterns from raw data. These patterns can then be used to gain a better understanding of a variety of factors and influence decision making. Data science does more than just crunch numbers — it reveals the “why” behind your data.
Data science is the key to making information actionable by using massive volumes of data to predict behaviors and infer meaning from correlating data in a meaningful way. From finding the best customers and charging the right prices to allocating costs accurately and minimizing work-in-progress and inventory, data science is helping businesses maximize innovation.
Data science tools and technologies have come a long way, but no development was more important than the improvement of artificial intelligence (AI). AI is the ability of computers to perform tasks that formerly were exclusive to humans. AI used to rely entirely on human programming, but thanks to the application of machine learning, computers can now learn from data to further develop their abilities. As a result, AIs can now read, write, listen, chat and even listen like a human can – though at a scope and speed that far exceeds what any one person is capable of doing.
Working together, these technologies are what enable data scientists to quickly and accurately make these groundbreaking determinations that influence countless business decisions. Data is constantly generated by the Internet of Things (IoT); data scientists develop machine learning and AI algorithms to understand the data and gain new insights; then, businesses deploy cutting-edge platforms to continuously monitor, evaluate and ultimately act on data, thereby adding value to many business processes and outcomes.
Starting your journey with data science can feel like an overwhelming undertaking, but when strategized and prepared correctly, it doesn’t have to be. Data science initiatives can deliver significant value to enterprises when the right opportunities are identified and new talents and technology are explored.
To help you get started, here is a breakdown of how data science and AI can unlock your business’s potential and get the most out of your most valuable asset.
How Data Science Can Impact Your Business
Data science can positively impact many business functions, both customer-facing and internally. And while the benefits and potential uses of data science are vast, here are some of the primary ways organizations have used data science in their operations, and the solutions they are using to get results.
Quantifiable & Data-Driven Decision Making
This is arguably the biggest reason many businesses utilize data science applications, and its usually also the biggest benefit. When organizations can organize, make sense of, and leverage their data, they can make more accurate predictions, forecasts, and plans for all areas of their operations. Using data science tools, businesses can determine what elements they need to focus on to reach their most important targets and can then implement the most effective plans to reach them. One relatively new but exciting feature of this technology is the ability to analyze streaming data through time series analysis, giving businesses real-time feedback that they can act on.
Better Understanding of Customer Intent
Organizations can now use data science tools to more effectively and accurately understand customer intent and their data, thanks in large part to what is known as natural language processing. Otherwise known as NLP, natural language processing utilizes AI to read, write, understand, and ultimately extract meaning from human language to make decisions. This is a major advancement for artificial intelligence and is changing the game for businesses and data scientists. Using NLP, they have expanded capabilities such as topic modeling, name entity recognition, and sentiment detection, all of which can help them more effectively utilize their data and understand their customers.
Recruiting
Recruiting and retaining quality and skilled employees is a struggle for many businesses, regardless of industry. NLP is also making a difference here, by automating aspects of the recruiting process to help organizations find better candidates, faster. Using unique algorithms, data science can “read” resumes and decide whether or not a candidate is worth pursuing. It can even select resumes based on specific character and personality traits, which enables businesses to get very specific about the type of person they are looking to hire.
Opportunity Identification
Another capability of data science tools and analytics is opportunity identification. Using historical and forecasted market data, businesses can identify geographic areas to target to penetrate for sales and marketing initiatives with greater accuracy. Data can inform new market decisions and make predictions as to whether a new venture is likely to be cost effective. This will ultimately help organizations determine what is worth the investment and whether they can expect to see a return.
Industry Opportunities with Data Science
Data science has far-reaching impacts on businesses across a variety of industries. To give you a better understanding of how different organizations are leveraging the benefits of data science, here are some use case examples from four major industries.
Data Science in Healthcare
The promise of big data in the health industry has never been more attainable. From performing predictive analytics during diagnosis to transforming treatment and improving patient outcomes, data science has never been bigger than it is right now in healthcare. In fact, McKinsey estimated that big data initiatives in the US healthcare system “could account for $300 billion to $450 billion in reduced healthcare spending or 12 to 17 percent of the $2.6 trillion baselines in US healthcare costs.”
Here are some examples of data science-driven healthcare innovations:
- Genomics and Precision Medicine: Using machine learning programs to analyze data from single cell sequencing, biomarkers, and genetics, providers have been able to develop and innovate more effective drugs at a faster rate.
- Clinical and Claims Data: Providers are improving patient outcomes and cost effectiveness by leveraging and analyzing markers from readmission predictions, efficacy and comparative analytics, prescription adherence, and market access analytics.
- EHR Data Usability: Using NLP, health care providers can read and act on electronic health records faster and more effectively. NLP can analyze, scan, and organize EHR interfaces into sections, making it easier for clinicians to find buried data and make diagnoses they might have otherwise missed.
Data Science in Manufacturing
Manufacturing in today’s digital world is all about staying one step ahead. From predictive process control and supply chain forecasting to predictive maintenance and image classification, leveraging data is the best way for modern manufacturers to keep up with industry competition.
Here are some examples of how data science is being applied in manufacturing:
- Supply Chain Forecasting: By utilizing supply chain data, such as transaction data, demographics, purchasing history, and trends, retailers can reduce inventory cost and maximize supply-chain optimization.
- Predictive Maintenance: No manufacturer can afford unexpected downtime, but with data science they can reduce failure rates and forecast maintenance using risk sensor stream data.
Data Science in Retail
For retailers in the digital age, data is everything and everywhere — shoppers and customers generate 2.5 quintillion bytes of data each day. From personalized offers to optimizing merchandise and inventory to next-generation store design, leveraging data to innovate is the status quo.
Some examples of the benefits of data science for retailers include:
- Real-Time Pricing Optimization: Retailers can use data science to optimize pricing based on consumer engagement, including historical sales data, price scheduling, and segment-level price changes.
- Data-Driven Stock, Inventory, and Ordering: Retailers can improve inventory optimization based on data from demand plans, forecasts, sales history, trends, and even local events/weather patterns.
- Trend Forecasting: Using NLP, data scientist can “scrape” online content to discover trending topics and likeability associated with those topics. Through topic modeling, retailers can then rank trends by likeability and use this data to inform purchasing of new inventory.
Data Science in Financial Services
From financial modeling to detecting risk and fraud to performing customer and credit analytics, leveraging data to innovate is a mainstay of the financial services market. To keep up with industry standards and competitors, many firms are turning to data science and machine learning. Data science helps firms extract knowledge from their data so that they can make fact-based business decisions, all while protecting sensitive customer information.
Some examples of data science and machine learning applications in financial services include:
- Credit Analytics: Firms can analyze risk and compliance management data, including customer relationship management system data, credit, risk, merchant records, products, and services.
- Customer Analytics: Transcription of calls between customers and financial service employees can be analyzed through sentiment detection to provide the organization with insight regarding which customer service team members has the most positive and/or negative interactions with customers.
Artificial Intelligence Impact in Business Today
Artificial intelligence may still sound like future technology, but it’s been a regular fact of life since the 1990s. AI was first used in simple things like chat-bots, speech recognition software, and even the Furby doll. A 2018 Harvard Business Review study found that the most meaningful way AI benefited businesses wasn’t in revolutionary breakthroughs, but in enhancing business processes on a day-to day level.
In the past few years AI has only become more powerful, more prevalent, and more capable of performing valuable tasks. Today, thousands of businesses — in sectors from manufacturing and retail, finance to healthcare — employ AI in three different applications: process, insight, and engagement.
Process applications
A process application is the automation of a simple task, such as extracting data, cross-checking information, and performing calculations. AI can automate labor in a fraction of the time that a human could perform, freeing up employees to perform more high-level tasks and be more effective at their work. Some common process applications include:
- Calculating optimal schedules: Businesses can determine the best time for deliveries, more effective appointment scheduling, and, through the use of a Computer-Aided Facilities Management (CAFM) system, can develop optimal schedules for employees.
- Enabling real-time decision-making: Customer service and administrative personnel can more easily pull up fully detailed customer histories, employee records, full inventory stock, future schedules, and even expected weather delays on-demand as the required data is pulled in fractions of a second.
- Delivering custom reports: Rather than having to constantly run reports manually, AI can assist in running periodic reports. Delivered reports can automatically vary based on when they’re run, who they’re run for, which metrics are relevant, and if they contain sensitive or confidential information. Powerful cloud-based AIs can run massive reports within minutes, instead of over hours.
Insight applications
An insight application is similar to a process application, except that it is also designed to detect and learn from patterns. This is where NLP and Optical Character Recognition (OCR) are used to analyze human writing and speech, and Computer Vision is used to process images and video to better categorize information. Insight applications can also use data to predict outcomes, providing users with an idea of what may happen in the future. Insight applications are capable of:
- Analyzing records: Private and public information can be analyzed in order to develop a better understanding of a defined element, from an individual person to entire communities. Social media can be reviewed for preferred shopping preferences and media consumption. Healthcare records and doctors’ notes can be analyzed to make better diagnosis.
- Personalizing content: Once there’s an understanding of a person’s preferences and lifestyle, businesses can generate content that specifically meets individual needs. Media recommendations can be tailored to interests. Offers and promotions can target those most likely to purchase. Insurance and financial providers can create specific models to make more calculated risks.
- Identifying and predicting: NLP has advanced to a point where it’s applicable to all industries. NLP tools can now identify instances of fraud, fake news, sarcasm, and even sentiment. Models can also be utilized to predict possibilities of fraud or other specified elements, allowing businesses to take action pre-emptively before real harm is caused.
Engagement applications
An engagement application takes processes and insights and uses that information to engage directly with humans. These types of AI applications can nearly mimic human conversation, or at least communicate in a way that allows for easier and more intuitive use of a program or service. Engagement applications are used for:
- Chat bots: These “conversational agents” can serve as the first point of interaction for potential customers. Whether on a website or standalone app, chat bots can answer simple questions, direct to resources, or collect information that will then be sent to a human agent.
- Employee resources: Businesses can develop internal information resources that employees can use to answer specific questions about work procedures, IT issues, or access HR support. Chatbots can also be used to streamline the onboarding process, saving time for managers and supervisors.
- Assisting diagnoses: Healthcare chatbots can gather information from people about symptoms and recent history, provide them with educational material, and offer updates on scheduling and appointments. This can assist doctors with making speedier diagnoses and can assist patients in remote locations.
Artificial Intelligence in the Future
AI has found use in all industries and services and will continue to find new applications in private industry, education and research, and in government. In particular, the industries of healthcare, finance, education, media, and customer service are expected to employ AI in even more powerful and pervasive ways.
The use of AI has been proven to save time and money through increased efficiency and optimization. As AI becomes faster and more scalable, those improvements will only continue — more time saved and better automation, plus optimization processes are forecasted to increase cumulative GDP by 16% by 2030.
The power and efficacy of NLP is expected to increase, bringing further advances in accuracy, multilingual models, and ease-of-use. Greater predictive capabilities will further advances in healthcare and manufacturing. Personalization of services and targeted messaging will become more precise for lifestyle, preferences, and circumstances.
As AI becomes more powerful and pervasive, businesses need to consider the impact on how their practices can affect customers, employees, and society. These ethical considerations include:
- There has to be an understanding of bias. Whatever parameters are put into an AI system will determine the information that comes out of it. Too often this can result in overlooking marginalized cultures, underprivileged communities, and minority populations.
- Privacy and personal information need to be protected and secure. Users should be provided options in what information they’re required to provide. While de-identification models can be adopted to assist in hiding identity, there needs to be an understanding that models are not 100% effective. Plans to prevent and repair data breaches need to be in place.
- There needs to be transparency on the potential replacement of the workforce. While a full automation of manual labor is not likely, as many as 2 million manufacturing jobs could be lost by 2025. Jobs in journalism, transcription, and research are also increasingly at risk at being replaced by NLP AI.
Getting Started with Data Science and Artificial Intelligence
The good news is that leveraging data science and AI is just a couple of steps away. The most important thing any business can do to prepare to implement data science initiatives is to organize their data. Unstructured data is a problem for 95% of businesses and prevents them from making more informed decisions across their operations. The first step is to gather as much data as possible — the more, the better — which will help prepare the data for data analytics and data science solutions.
Then businesses need to consider what, exactly, they want and need out of an AI system. To get started with employing an AI system, businesses need to determine their Key Performance Indicators (KPIs) to establish their strategy going forward. The important steps are:
- To identify their goals and prioritize which take precedent. Is it more important to boost profitability or improve customer satisfaction? Would it be better to minimize risk or maximize profits?
- To recognize that nothing is perfect, and that includes AI. Businesses will need to establish a threshold of “good enough” probability and determine what are acceptable levels of false positives in reports and predictions.
- To understand that they’re already sitting on a wealth of established data. Rather than looking for new means of information gathering, the better question is, what can be done to make the most of the data they already possess?
- To consider how their data could be enriched using available public data. Potential resources include NOAA for weather, Yahoo Finance, Census and Zoning data, and the 2021 Hospital Price Transparency Rule.¬¬
Once we help you compile your data and outline your AI strategy, it’s time to hand it over to a trusted team of experts, who can move the data to a cloud format, where it can be analyzed and ultimately leveraged by your organization. At Hitachi Solutions, we have been helping businesses from a variety of industries put their data to work for them. Our accessible and effective solutions can be tailored to any operation and any goal. If ¬¬you’re ready to make the most of your data, contact us — we’re ready to get started.