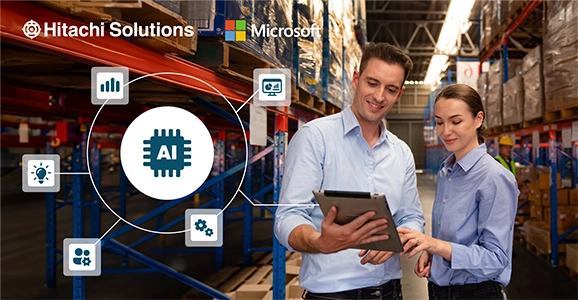
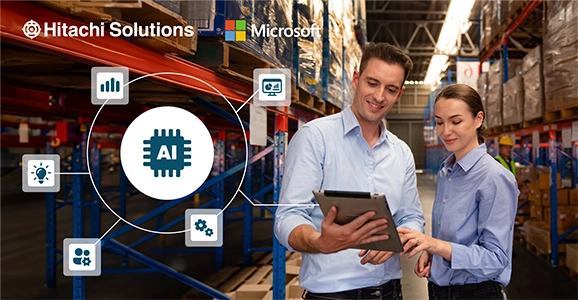
Are you ready for the “Age of AI”?
Artificial intelligence, in general, can be thought of as a technology to augment or automate decisions. But what makes AI so interesting, especially compared to most other technologies out there, is how ubiquitous it is in its application. It impacts nearly every industry, nearly every department, and even every job function – from the shop floor all the way up to the CEO.
In fact, I have no doubt in my mind that it will eventually be as ubiquitous in its application and reach as the internet (or even electricity), and quickly become integrated in most things we do in our professional and personal lives.
But while AI – and its subfields, such as generative AI – offers incredibly innovative opportunities for every manufacturing department, a measured, test-and-learn approach is advised to balance potential gains with its inherent risks and challenges.
Let’s take a closer look at generative AI, ChatGPT, and what manufacturers should be doing now to get started.
How Does Generative AI Fit into the Field of AI?
Artificial Intelligence (AI) is the overarching discipline that covers anything related to making machines and computers behave intelligently. This includes basic rules-based systems and more complex systems that mimic human intelligence.
Machine Learning (ML) is a subset of AI that involves the practice of using algorithms to parse data, learn from it, and then make predictions or decisions without being explicitly programmed to do so. The learning occurs when the machine or model becomes better at decision-making as more data is analyzed and more feedback is provided.
Deep Learning is a subset of Machine Learning inspired by the structure and function of the human brain. It employs neural networks with several “hidden layers” (deep neural networks) between the input and output layers. These hidden layers perform computations and pass the information from one to the next, allowing the system to learn complex patterns in the provided data.
Generative AI is a further subset of deep learning. Generative models are capable of creating entirely new data that resembles the training data. For instance, generative AIs can create realistic human faces or write human-like text. They do this by learning the probability distribution of the input data and generating new data from the same distribution, hence “generating” new, unseen content.
ChatGPT is a type of Generative AI developed by OpenAI that utilizes the GPT (Generative Pretrained Transformer) AI model. By predicting the next word in a sequence given the previous words, it generates human-like text responses instead of selecting from predefined responses. This system integrates aspects of AI, machine learning, deep learning, and generative AI.
Let’s Take a Closer Look at Generative AI and ChatGPT
Generative AI
Generative AI is a subfield of artificial intelligence (AI) that focuses on creating new content, data, or solutions autonomously by learning from existing data. It leverages machine learning techniques, particularly deep learning and neural networks, to generate outputs that resemble real-world examples. This technology has a wide range of applications, such as generating art, creating realistic game environments, data augmentation, drug discovery, and even enhancing privacy by generating synthetic datasets.
It has significantly contributed to the advancement of AI by enabling more creative and diverse solutions across various domains. Popular generative AI models include Generative Adversarial Networks (GANs), Variational Autoencoders (VAEs), and Transformer-based language models (e.g., ChatGPT).
Transformer-based language models, like GPT-4, are a class of generative AI models that employ the transformer architecture to excel in natural language processing (NLP) tasks. Transformers use self-attention mechanisms to capture complex dependencies within textual data, enabling them to generate contextually relevant outputs. These models are pre-trained on large text corpora, learning patterns and structures that represent the grammar, syntax, and semantics of a language.
Once trained, transformer-based models can be fine-tuned for various NLP tasks, including text generation, where they generate coherent and contextually relevant text based on a given input or prompt. Notable transformer-based language models like OpenAI’s GPT series (GPT, GPT-2, GPT-3, GPT-4, and ChatGPT) and Google’s BERT have demonstrated impressive performance in both generative and discriminative NLP tasks.
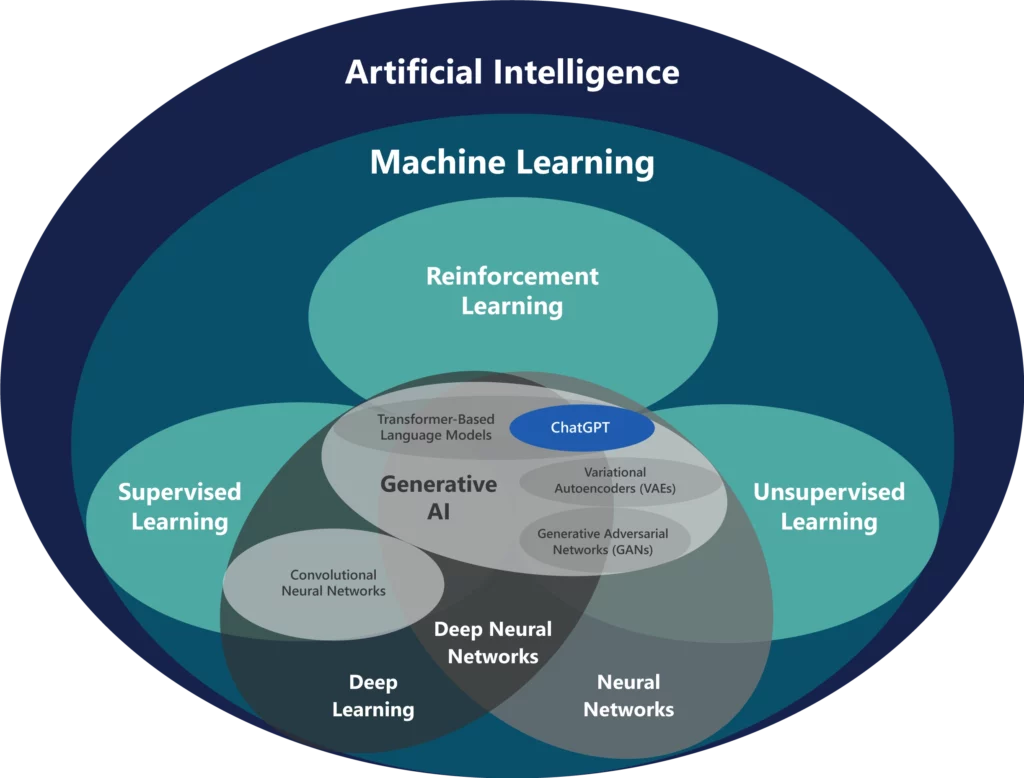
ChatGPT
ChatGPT is the famous AI language model developed by OpenAI that can generate human-like text based on the prompts it receives. ChatGPT really brought the technology of AI – and specifically, generative AI – into the hands and the vernacular of most the world.
If you think about it, ChatGPT was most people’s direct experience with AI capabilities. Because ChatGPT became the popular AI product, the term has now become the verb to represent generative AI – similar to how we say “Google it” when representing searching the internet.
Here’s how should you be thinking about ChatGPT:
ChatGPT is like a fast-food drive-thru for information: it’s quick, it’s convenient, and it usually gets the order right, but don’t be surprised if you find a few unexpected things in your bag.
So treat it like having an AI-powered personal assistant at your fingertips – it can handle a lot of your daily paperwork, respond to emails, draft presentations, and look up information. But like any assistant, it doesn’t have the context or business acumen that you possess – it needs someone to direct it and to check its work.
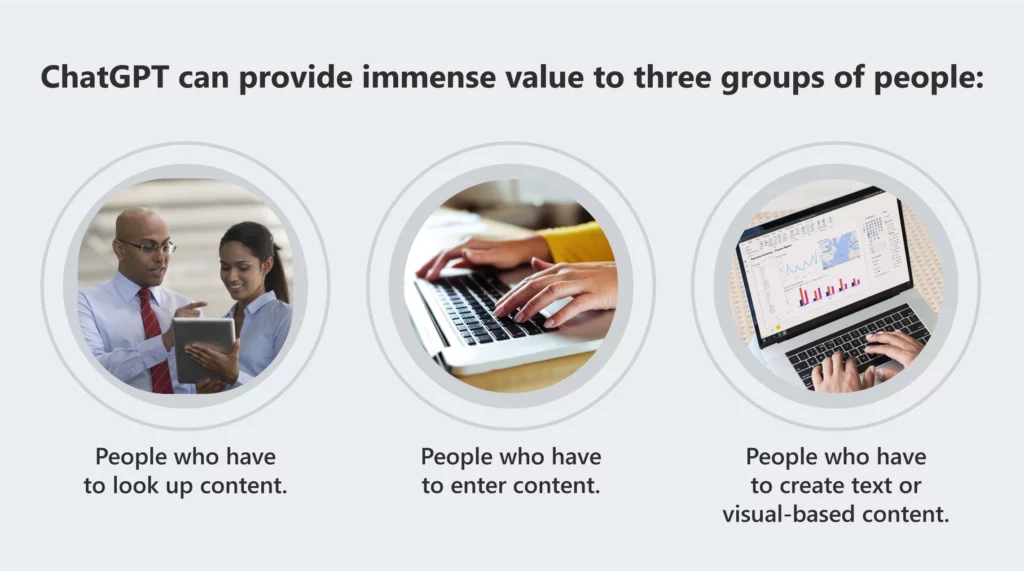
The First Step: Create a Company Policy and Strategy for Generative AI
While generative AI offers incredible, innovative opportunities for every manufacturing business department – especially for marketing, customer service, product management, and software development – a measured test-and-learn approach is advised to balance the potential gains with the inherent risks and challenges.
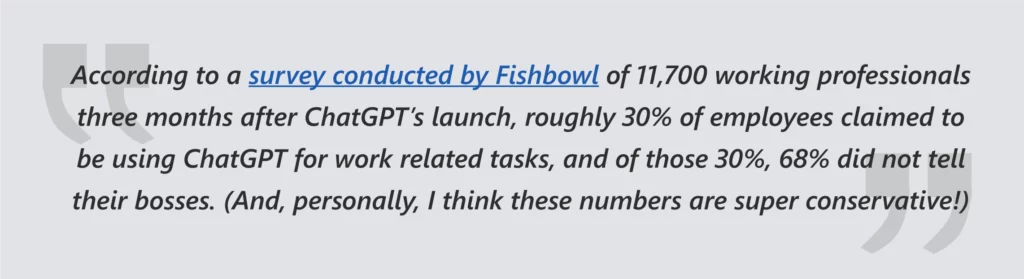
The point is that a lot of people are using ChatGPT, but are they using it correctly or incorrectly? Are they relying on it when they shouldn’t? Or worse, are they unknowingly exposing company information to the public?
The first step for every company, regardless of industry or digital maturity, is to come up with both a policy and a strategy for generative AI. Here’s what I mean by the two terms:
- A policy is any established rules, guidelines, and standards that govern behavior in a company.
- A strategy is an approach for achieving long-term goals and competitive advantage by providing direction and defining key actions for success.
Three Key Areas Where You Need Generative AI Strategies and Policies in Place
1. Open Generative AI Tools
Your employees are using ChatGPT – do you have rules around what they can use it for? What company information are they allowed, or not allowed, to load into it? A lot of people don’t realize that the minute they load something into the public version of ChatGPT, that data is used to train the company’s algorithms – which means that it’s potentially available and accessible to anyone.
Also, what kinds of answers are your employees relying on it to provide? ChatGPT will always give you an answer, but it may not always be right. For instance, asking ChatGPT to summarize an article has much lower risk, with much lower implications, than asking ChatGPT to do calculations.
2. Generative AI Tools Integrated into Business Applications
Microsoft’s integration of the OpenAI platform into its suite of products – branded under the name Copilot – means that ChatGPT’s capabilities can be turned on in Microsoft applications with the proverbial flip of a switch, and nothing needs to be done on your end. When they are available to your employees, how are you going to make sure they’re using them productively (because, let’s face it, they are fun to use!), and also that they’re using them in alignment with company policies?
Let’s use Microsoft Teams as an example. When you record a meeting in Teams, you can make the meeting transcription accessible to the ChatGPT model that’s built into Teams and ask it to 1) summarize the meeting in less than 150 words, and 2) list out all the assigned action items that were covered. This is a really cool feature, but you have to record meetings in order to do it. Maybe there isn’t any issue with employees recording internal meetings, but what is the policy around recording external meetings with customers, partners, or suppliers?
3. Private Generative AI Tools
Microsoft’s Azure OpenAI services means that companies have access to the ChatGPT model and can build their own enterprise ChatGPT inside their company, where they can safely and securely attach their own company data to it. You can do some really transformative things with this, but you need strategy and policy to support it.
A private enterprise ChatGPT can be divided into internal use and external use:
Internal use is where you’re going to rely on your own ChatGPT to make decisions for you and your employees. But a question you need to answer is, how are you going to validate the fine-tuning of your model’s output (especially in the case of calculations or forecasts)? Is validating the output two times good enough? Or do you need to validate it 100 times? You need a solid answer to that before moving forward.
External use is where you use your ChatGPT as an interface with customers – such as for support, or customer service, or even for sales. So think about it like a chatbot on your website, but on steroids.
For instance, imagine you’re a retailer like Nordstrom. As a customer, you could type into the “Nordstrom GPT” something like, “I really like the style of Pierce Brosnan in The Thomas Crown Affair – what do you have that you could recommend that would look like him?” Having a “company ChatGPT” that can interface with customers and provide personalized recommendations dramatically changes the way companies engage.
Hitachi Solutions’ Enterprise Chat offers a private generative AI experience that is designed to boost internal productivity, improve customer experiences, and reduce operational costs – all while keeping your data confidential and secure. But if you’re not sure where to start, our two-week assessment can help guide you toward how to prioritize and prepare for leveraging AI as a solution tool in your organization.
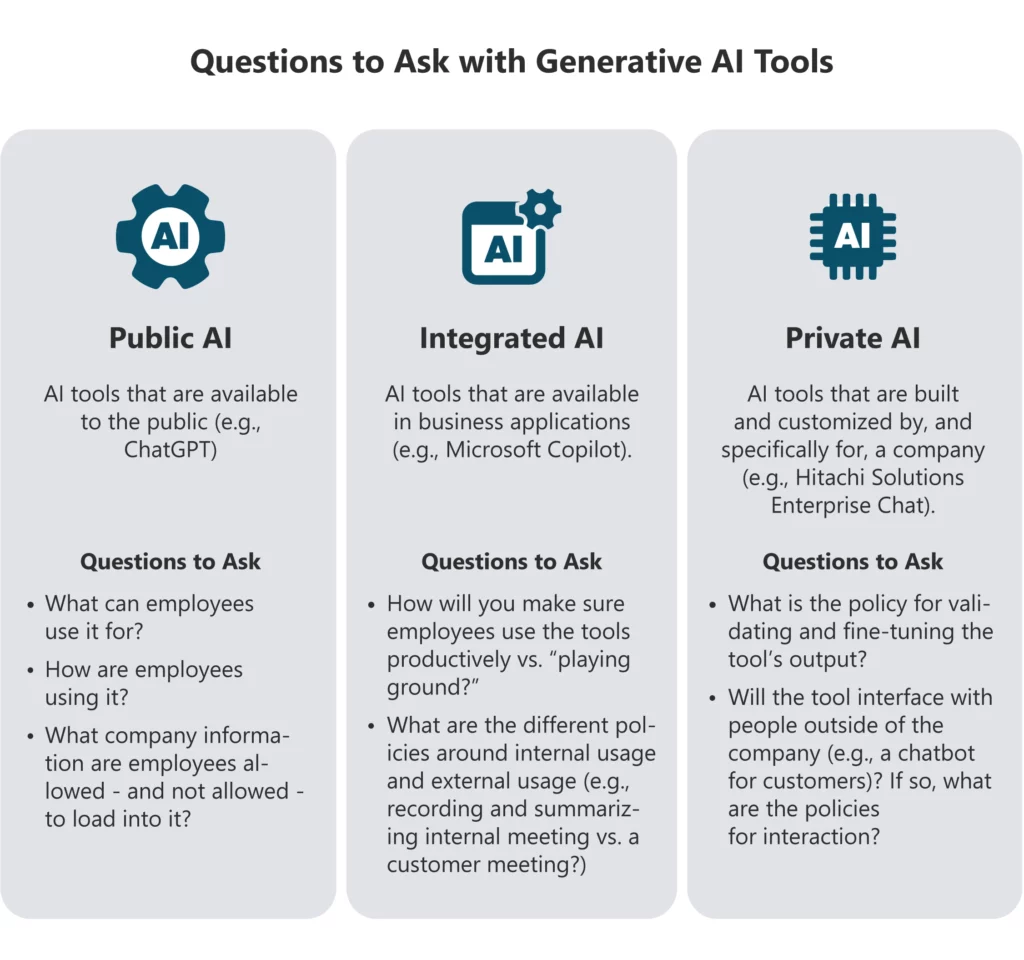
Where Do You Start with Defining Company Policy and Strategy?
So getting a company policy and strategy in place are critical, but where do you start in defining them? The first exercise I like to do is the “executive knowledge test,” which is asking your senior business leaders this one question:
“If all your company’s data were accessible and loaded into your own ChatGPT, what would be the top three things that you would ask it?”
I love this question because it shows intent and vision, as well as an understanding of the technology at the same time.
What I’ve found from personal experience, when it’s done, that roughly 25% of the questions are roughly not possible or practical to answer, or they are terrible uses of ChatGPT. Those kinds of questions show a lack of understanding in how the technology works. Questions like, “What company should I acquire?”, or, “Based on our business, where should we vertically integrate?”
Roughly 25% of the questions are perfect uses. Things like a CFO saying, “Here’s a list of our top 10 competitors – compare their 10-K reports and identify areas of similarity and difference.” Or a CMO asking to summarize and categorize customer sentiment based on survey responses, reviews, and even social media tags.
But the main bulk of questions – 50% – have nothing to do with ChatGPT. The answers to those questions are determined by completely different technologies, and in some cases, are things that we’ve been able to do for arguably decades. For example, a COO asking, “What’s my current demand forecasting accuracy?”, or a Chief Revenue Officer saying, “Predict my future revenue for the month of July.” Yes, ChatGPT could pull the answers, but the answer comes from somewhere else.
Ultimately, the executive knowledge test helps highlight the things that a company wants to use ChatGPT for that may or may not be truly leveraging the power of what it can do. This usually results in business leaders having a much larger conversation around what ChatGPT can actually do, what they want it to achieve, and where they should be putting their effort.
Leading in the Age of AI
Just as AI learns from us, we must learn from it; adaptability in the Age of AI is not optional, it’s survival.
Artificial intelligence, in various forms, has been intertwined with daily life for several years. However, the rapid proliferation of ChatGPT and other conversational and generative AI models has made this technology easily accessible to the general public. This accessibility shows a glimpse of AI’s power and potential, which inspires some and scares others.
But the bottom line is that if you’re not developing a strategy for how your business can securely and responsibly harness the power of AI, you’re going to quickly fall behind. Contact us for guidance on how to take the next step in your AI journey, and which areas of your business can gain the most value.