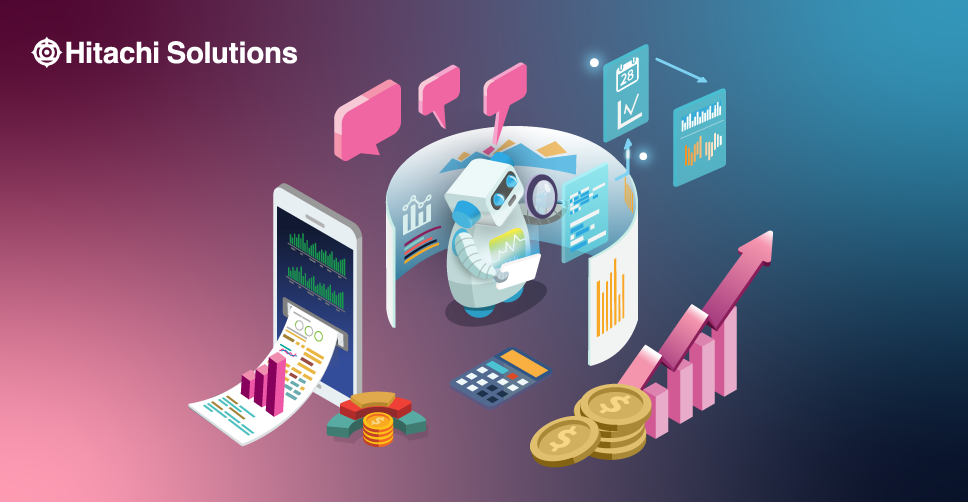
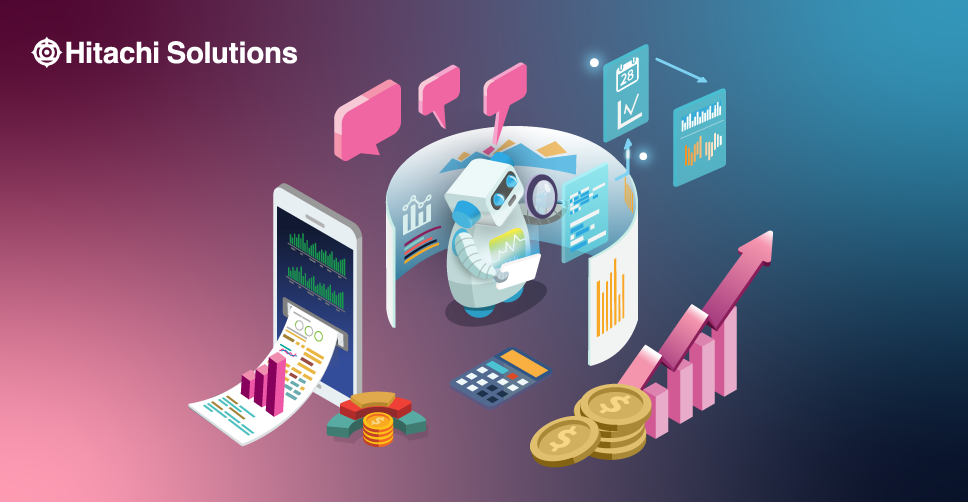
Natural Language Processing Lab
Unlock access to text analytics in 4 weeks
Download the OfferWhen people think of natural language processing, artificial intelligence typically comes to mind, and for good reason. NLP is a subset of AI, one that leverages machine learning algorithms to enable computers to understand and interpret human language. NLP is most often associated with efforts to optimize human-to-machine communications — think, a customer service chatbot or even an at-home virtual assistant like Alexa. But this is just one application of NLP, and it barely scratches the surface of what this incredible technology is capable of.
Many different industries use NLP to great effect; in this blog post, we’ll focus specifically on natural language processing applications in the financial services sector.
The 5 Natural Language Processing Applications You Need to Know
Though there are many different applications for NLP in the financial services space, five in particular stand out:
- Optical Character Recognition (OCR): According to TechTarget, OCR refers to the practice of using technology to “distinguish printed or handwritten text characters inside digital images of physical documents.” The most common application of OCR is converting physical documents into an electronic format — for example, scanning a paper document and turning it into a PDF. OCR is sometimes also referred to as text recognition.
In regard to NLP, OCR is used to scan unstructured datasets (such as images, PDF, text files, and so on), extract text and tables from that data, and present it in a digestible format so that it can be fed into an NLP pipeline for further analysis. - Sentiment analysis: Sentiment analysis is a technique used to determine the underlying sentiment for a piece of writing — that is, whether it expresses a positive, negative, or neutral sentiment. Also known as sentiment detection or opinion mining, sentiment analysis relies on a combination of NLP, text analysis, computational linguistics, and biometrics. Businesses frequently apply sentiment analysis to social media to gauge public opinion about their products, services, or brand as a whole.
- Topic Modeling: Topic modeling is a form of statistical modeling and NLP used to classify collections of documents. It achieves this by scanning documents, detecting common words and phrases, and grouping them together in order to identify semantic structures, or “topics.”
One of the most popular methods of topic modeling is latent Dirichlet allocation (LDA), which is a “generative probabilistic model for collections of discrete data, such as text corpora.” In layman’s terms, LDA is an algorithm that recognizes semantic relationships between different words and groups them accordingly. - Text Classification: Text classification describes the use of NLP to analyze text data and assign tags or labels to different text based on predefined categories. For example, an organization might use text classification to sort incoming customer support tickets based on whether they include certain key words or phrases, such as “complaint” or “refund,” so that they can then be routed to the appropriate representative. Text classification is also commonly referred to as text categorization or text tagging.
- Named-entity Recognition (NER): Also known as entity chunking, entity extracting, or entity identification, NER is a form of information extraction often used to segment named entities into predefined categories. For reference, a “named entity” is a real-world subject, such as a person, location, organization, or product.
Benefits to Using Natural Language Processing in Financial Services
Now that we’ve defined the five most important natural language processing applications, let’s talk about how firms in the financial services sector can benefit from using them.
First and foremost, NLP can be used to perform investment analysis — that is, to gather summary insights of market intelligence in order to get a better read on what’s happening in the market. This is typically achieved by using sentiment analysis to scan news reports, social media posts, and other publications to identify public discussions about firms and inform future trades. Firms can use investment analysis as a feature in predictive modeling to anticipate future performance of different securities or industries and develop investment strategies based on that information.
Keying off of that, financial services firms can also use NLP to assess the competitive landscape through environmental, social, and governance (ESG) ratings. ESG ratings are typically used to help investors evaluate companies in which they might want to invest; a high ESG rating is positively correlated with the valuation and profitability of an institution, whereas a low ESG rating is negatively correlated with volatility. Using sentiment analysis, firms can compute their ESG rating, as well as those of their primary competitors; this enables them to gauge the overall health of their organization, as well as its status relative to others in the market.
NLP can also be used to ensure compliance. The financial services sector is certainly no stranger to regulation, nor to the substantial penalties associated with non-compliance. Firms can use text classification and NER to determine whether their traders are compliant with relevant rules and regulations by analyzing corporate data and flagging certain key words. Thanks to OCR, firms can even apply text classification and NER to large batches of unstructured data and use those results for topic modeling.
In much the same vein, NLP can help detect money laundering and fraudulent activity, both of which are major cost centers for financial services institutions. Again, a firm could utilize OCR to convert unstructured data into a usable format, then apply text classification and NER to tag certain words associated with fraudulent behavior. The firm could then build topic models to synthesize text and identify the main ideas or topics within that collection of documents. Finally, the firm could employ sentiment analysis, text classification, NER, and topic modeling as features in a predictive model.
Financial services firms can also leverage NLP to better understand their customers. Using OCR and sentiment analysis, firms can scan through customer comments, reviews, social media interactions, phone log transcripts, and more and pull out contextual and behavioral data. Armed with this information, firms can gain valuable insights into customer behavior, optimize customer experience, and even predict future actions and purchases.
As you can see, there’s significant overlap between each of the five natural language processing applications in financial services outlined above; that’s because different NLP techniques work best when used in conjunction, enabling firms to get greater mileage out of this innovative technology.
Hitachi Solutions Helps You Do More with Your Data
If you’re looking to do more with your data using natural language processing, Hitachi Solutions can help. We offer a range of NLP solutions across various applications — including the five shown above — to help financial services institutions such as yours uncover hidden insights. Best of all, Hitachi Solutions is equipped to provide NLP services with unmatched scalability, speed, and accuracy.
Take of this exciting technology today — contact us for more information.