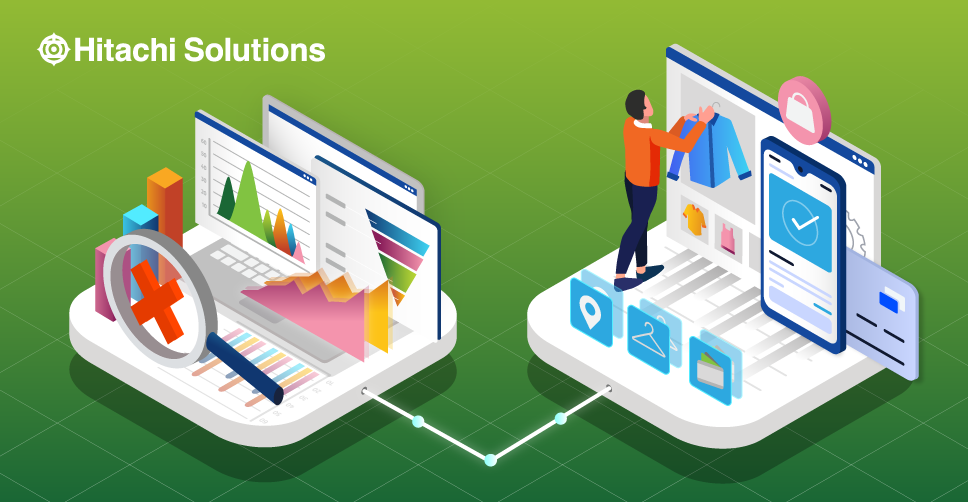
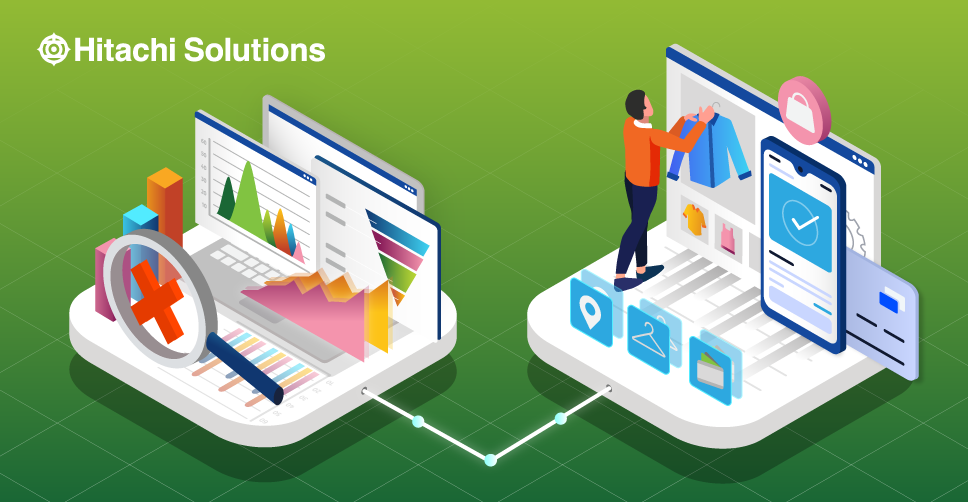
Building a Clear View of Your Customers
The infographic highlights the key challenges faced by businesses as they create a customer view through unified data, analytics, and more.
Download the InfographicTo say that Big Data is having a moment in the retail industry would be an understatement. Big Data has been breathlessly hailed as a “game-changer” and the “future of retail” by countless industry publications, a powerful asset with transformative potential — at least when coupled with retail analytics technology and a solid data strategy.
On its own, Big Data is exactly what it sounds like: massive quantities of static data to sift through. But when processed and analyzed, Big Data comes to life, revealing valuable insights that enable retailers to deliver hyper-personalized customer experiences, optimize inventory and supply chain management, uncover new growth opportunities, and make more informed decisions across the board.
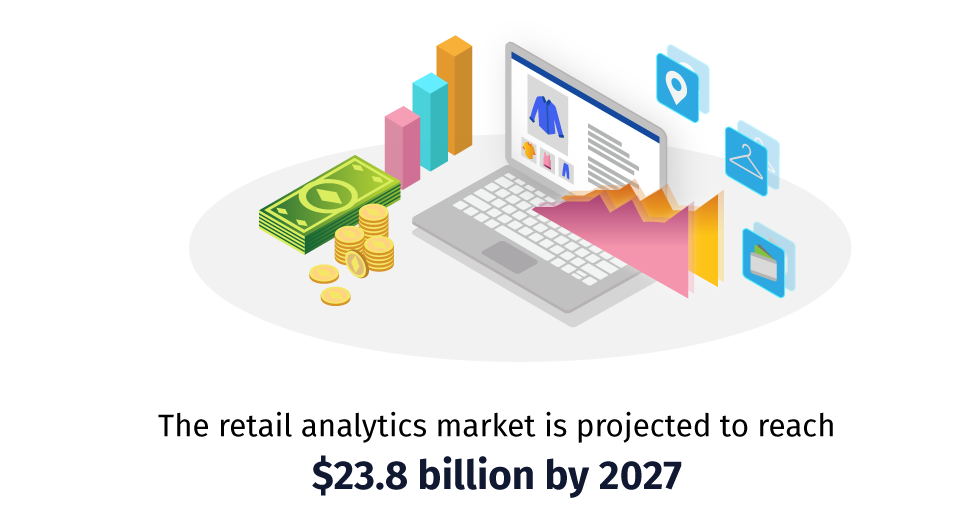
Given Big Data’s extraordinary potential, it’s little wonder that companies have made significant investments in retail analytics solutions — or, consequently, that the retail analytics market is projected to reach $23.8 billion in value by 2027. In order to maximize the value of that investment, retailers must also develop a data strategy — one that calls upon the expertise of data scientists, implements strong data management and governance policies and makes data not only accessible but actually usable by employees at all levels of the organization.
With these factors in mind, let’s dig deeper into the concept of Big Data and its innumerable applications in the retail industry.
3 Terms You Need to Know
Before we get into it, let’s take a step back and talk about what we really mean when we say “Big Data,” “retail data analytics,” and “data strategy.”
For starters, Big Data is a term used to describe large volumes of structured, semi-structured, and unstructured data. In the retail industry, the data that comprises Big Data can take many forms, including customer data, product data, sales data, inventory data, supply chain data, and so on. Certain types of data even have subcategories — for example, identity data, behavioral data, descriptive data, and qualitative data are all types of customer data. This data can come from internal sources, such as point of sale (POS) systems, or external sources, such as third-party companies.
Retail data analytics collectively refers to the techniques retailers use to gather, process, and analyze data in order to generate actionable insights and meaningful findings. There are four types of retail data analytics:
- Descriptive Analytics: Descriptive analytics is perhaps the most common form of retail analytics; it answers the question of “What happened?” by identifying patterns within historical data and placing things within their proper context. Common descriptive analytics techniques include traditional business intelligence and visualizations.
- Diagnostic Analytics: If descriptive analytics answers the question of “What happened?”, diagnostic analytics answers the question of “Why did it happen?” Essentially, diagnostic analytics aims to address the contributing factors that led to a specific outcome. Common diagnostic analytics techniques include drill-down, data discovery, data mining, and correlations.
- Predictive Analytics: Predictive analytics is all about making educated guesses about the likelihood of future outcomes based on historical data. An incredibly valuable form of retail data analytics, predictive analytics can be difficult to execute without good quality data and the support of an experienced data science team. Common predictive analytics techniques include regression analysis, forecasting, pattern matching, and predictive modeling.
- Prescriptive Analytics: The final frontier of retail data analytics, prescriptive analytics provides data-driven recommendations that are intended to help retailers make more informed decisions. Much like predictive analytics, prescriptive analytics is an incredibly advanced form of retail data analytics and requires considerable data science expertise to successfully execute. Common prescriptive analytics techniques include graph analysis, machine learning, neural networks, recommendation engines, heuristics, simulations, and graph analysis.
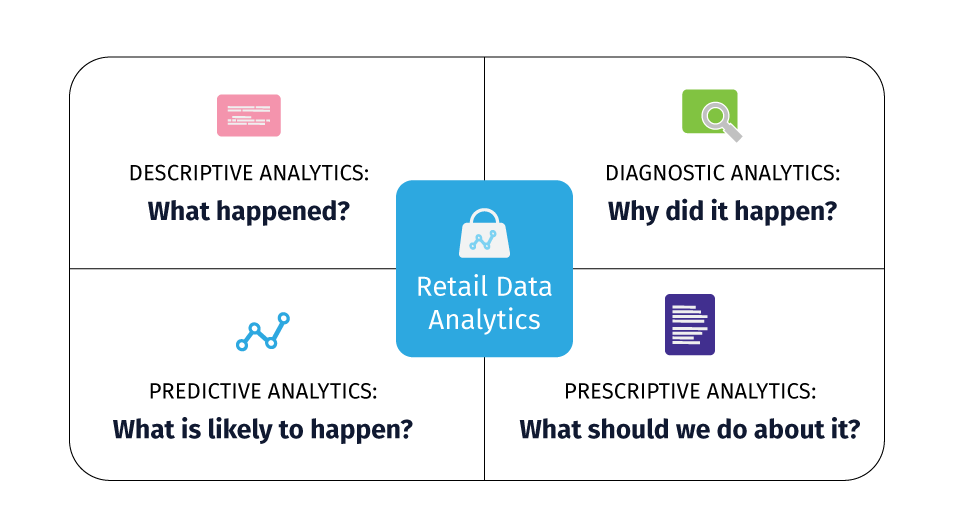
Last but certainly not least, a data strategy provides a roadmap for the application of advanced analytics in retail. In other words, your company’s data strategy sets clear expectations and requirements for data acquisition, storage, processing, sharing, governance, and analysis. It also ensures that any actions taken as a direct result of data analysis align closely your stated business objectives.
Let’s put it all together: In order to reap the rewards of Big Data, retailers require the right combination of retail data analytics technology and a comprehensive data strategy.
5 Benefits of Big Data in Retail
We’ve alluded multiple times now to Big Data’s potential — let’s talk more specifically about those prospective benefits. By combining retail data analytics technology with data strategy, retailers can:
- Take personalization to the next level. Customer data offers valuable insights into how customers think, feel, and behave, both at the collective and the individual level. Retailers can use retail data analytics to segment customers based on shared characteristics and build detailed profiles for individual customers based on which demographics they belong to, their purchase history and personal preferences, whether they’re enrolled in a customer loyalty program, and so on. Using this information, retailers can deliver hyper-personalized product recommendations, marketing campaigns, product discounts, and more, thereby creating a truly singular customer experience.
- Forecast changes in consumer demand. By applying predictive analytics to historical data, market data, demographic data, behavioral data, browsing trends, and more, retailers can generate demand forecasts with surprising accuracy. These forecasts can inform everything from advertising campaigns, to inventory management, to staffing decisions.
- Anticipate supply chain disruption. Companies can use retail data analytics to closely monitor disruptive events as they unfold, assess their potential impact on the business, and make strategic decisions about how to mitigate the effects of disruption. Retailers can even apply analytics to real-time data from external sources in order to anticipate disruption before it even happens and plan accordingly.
For example, let’s say you pulled reports from the National Oceanic and Atmosphere Administration predicting a severe hurricane season along the Gulf Coast. Based on that information, you might divert shipments from ports along the Gulf Coast during hurricane season, using prescriptive analytics to determine which ports you might reroute to without causing shipment delays. - Unlock insights from customer journeys. Customer journeys are more complex than ever, spanning multiple in-person and digital channels. Retail data analytics surfaces valuable data points from those journeys and analyzes them in order to reveal insights about customer behavior — for example, you might use analytics to determine:
- Which channels customers are most likely to use,
- Which touch points they’re most likely to engage with,
- Which marketing materials they’re most likely to respond to
- Which stages of the customer journey have the greatest impact on customer satisfaction
- At what point in their journey customers are most likely to convert
- And more
- Incentivize sales with optimal pricing. Companies can leverage advanced analytics to gauge how customers respond to different product pricing levels and predict how price changes will impact sales. This enables retailers to set competitive prices that maximize sales profitability and offer discounts or product bundles for less popular items, capturing lost sales and increasing inventory turn.
Common Retail Analytics Mistakes (& How to Solve Them)
Given the possible benefits it offers, it’s easy to get excited about Big Data. But all too often, retailers make the mistake of investing in an advanced analytics solution without a clearly defined data strategy to support it, or a plan for how to put the insights such a solution can provide into action.
Don’t miss out on the incredible potential of Big Data in retail — take a look at these common, costly mistakes companies make with retail data analytics and learn how to avoid making them yourself.
Mistake #1: Losing sight of the human element.
For many businesses, a retail analytics solution can seem like a shortcut to data-driven decision-making. Although it’s true that such a solution enables you to analyze massive quantities of data, it’s wrong to assume that technology alone can solve all of your problems — after all, what good is a state-of-the-art retail data analytics solution if no one in your organization knows how to use it?
It isn’t enough to simply invest in the right solution — your employees also need to understand how it works, how to interpret data, and how to put insights gleaned from that data to good use. This requires investing in adequate training for existing employees and looking for candidates with data science and analytics experience as you grow your workforce. By ensuring that your employees are comfortable with using retail data analytics technology, you can maximize your investment and give your employees the confidence to do their jobs more effectively.
Mistake #2: Thinking a “one-size-fits-all” solution is the answer.
In an ideal world, you’d be able to use a single retail data analytics solution across your entire organization. In reality, however, this “one-size-fits-all” approach is highly impractical. After all, your organization isn’t homogenous: It consists of multiple departments and hundreds — if not thousands — of business users, all of whom think and behave differently. Bearing that in mind, you’ll want to invest in a solution with the flexibility to accommodate this diversity of thought and action.
The best place to start is with a visualization service that makes data easily digestible by end-users, business analysts, and IT personnel alike. The next step is to implement tools and services that enable you to create detailed and highly formattable reports that provide a clear view into every level of your business’ operations and every stage of the sales process. Finally, look into a toolset that operates on a self-service model that’s easy to use and intuitive for members at all levels of your organization.
Mistake #3: Failing to define key metrics.
In order to get the greatest value out of your retail data analytics investment and to gauge the success of your data strategy, you must first define the metrics you intend to use to assess your business’ performance. These key performance indicators will act as quantifiable goals for your team of business analysts, flag areas for improvement, and ensure that any data-driven actions your company takes align closely with your stated business objectives.
If you’re unsure of which metrics to monitor, here are a few high-value KPIs to consider:
Mistake #4: Building an analytics culture from the bottom-up, rather than the top-down.
When it comes to developing their company’s retail data analytics culture, many prefer to take a bottom-up approach because this approach uses data as a jumping-off point and provides a more accurate forecast on which to base business strategy. There’s just one glaring issue with this approach: It forces you to tailor your business strategy to fit the data, which could run contrary to your company’s needs.
When it comes to choosing a retail data analytics solution, it’s best to take an outcome-driven approach. Start by identifying the problem you want to resolve, conduct a proof of value on that objective, confirm the results, and invest in technology accordingly.
In terms of building a retail data analytics culture, go top-down rather than bottom-up. A top-down approach prioritizes business initiatives, uses Big Data to strategically optimize operations, and motivates teams to work collaboratively to achieve a broader goal, eliminating data silos in the process.
Taking a top-down approach saves time, money, and effort by eliminating the need for extra tools and systems and enabling employees to dedicate their attention to the goals you’ve set. With the right solution, top-down retail data analytics can even provide a broad view of the market, enabling analysts to identify patterns and make data-driven decisions that propel business growth.
Mistake #5: Allowing data silos to form
Data silos are one of the biggest obstacles to taking full advantage of advanced analytics in retail. Silos can organically develop from divisions between organizational departments. Since each department has its own unique set of objectives, they can sometimes be hesitant to share data and resources, often at the expense of identifying valuable business opportunities.
If you’re experiencing issues with data silos, you’re not alone: One in four organizations have more than 50 distinct data silos. Even amongst organizations that consider themselves highly data-driven, 39% report having more than 50 data silos. Once they become entrenched in company culture, silos can be incredibly difficult to eradicate; that said, there are still measures you can take to mitigate the damage done by silos.
The best place to start is by creating a consistent interdepartmental methodology for handling Big Data. Rather than separate members of your retail data analytics team into different groups based on who collects, processes, and makes decisions with data, reimagine advanced analytics as a collaborative process. Most importantly, make sure to invest in an operational solution that offers seamless integration not only across departments, but also across systems in order to promote data and resource sharing, collaboration, and transparency.
Revolutionize Retail with Hitachi Solutions
Drowning in a sea of data? Hitachi Solutions is here to throw you a lifeline.
We’ve created a holistic offering that addresses your most pressing data challenges, so you can focus on what you do best: creating memorable products and experiences that delight your customers. Called Empower: Analytics as a Service, this is a fully managed, subscription-based service offering that provides the data cultivation, governance, and monitoring tools you need to ensure that your data lake doesn’t become a data swamp. Empower is designed to help your retail company operationalize intelligence for all and, at the same time, provide analysts and data scientists with fast access to semi-structured and unstructured data to drive actionable insights quickly and easily.
But that’s not all: We’ve also built an entire business unit dedicated to helping organizations derive valuable insights from Big Data. From data and analytics services to a dedicated data science team, we offer a vast library of resources and enterprise analytics expertise to help you revolutionize retail operations.
Start putting your data to good use — contact Hitachi Solutions today for more information.