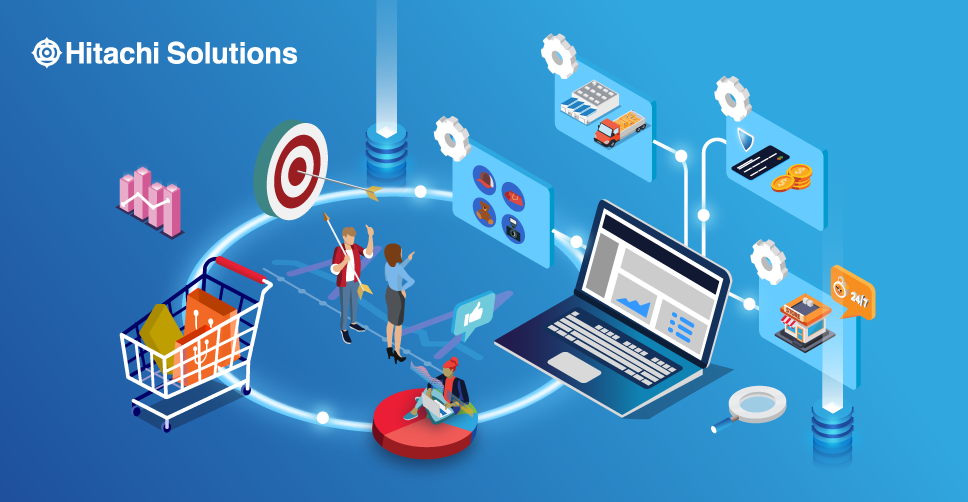
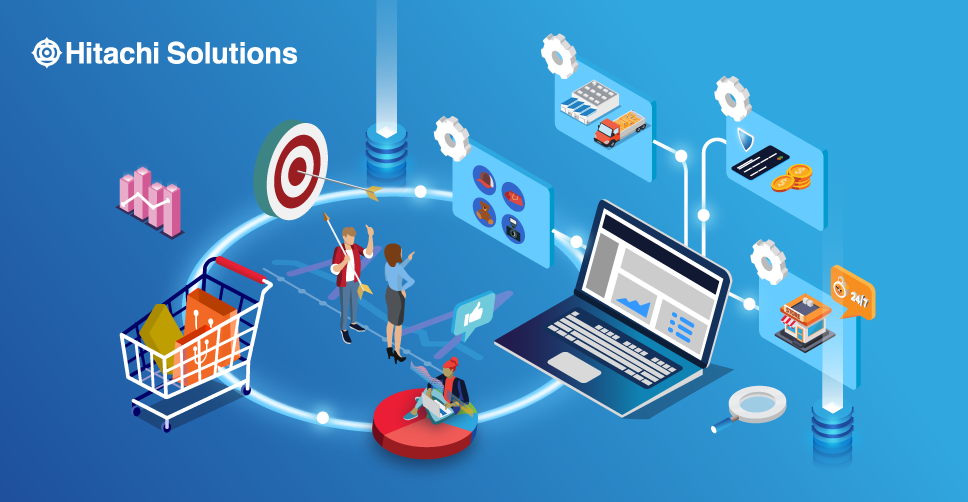
Customer Data Platforms: Solving the 5 Key Business Challenges in 2023
This comprehensive guide unpacks the potential of Customer Data Platforms (CDPs) in meeting key business challenges, providing you with in-depth case studies, insights on data governance, and a roadmap for responsible AI integration.
Download the WhitepaperRetailers need to understand their customers in order to succeed — a key fact that has led to the rise of retail data science. Big data analytics in the retail market was valued at U.S. $4.9 billion in 2020 and is projected to reach $26 billion by 2028, registering a compound annual growth rate of 23.1%. This growth is largely driven by:
- The need to deliver personalized customer experiences to increase sales
- An explosive growth in ecommerce accelerated by the COVID-19 pandemic
- The need to stay on top of industry trends and forecast potential supply chain disruptions
- The introduction of retail technologies powered by the internet of things (IoT), artificial intelligence (AI), and machine learning
Retailers must operate accurately in real-time while taking just-in-time advice from disparate datasets arriving from collaboration points across their entire value chain. The staggering number of channels adds to the difficulty of how to manage high volumes of fast-moving data in a cost-effective manner.
Thankfully, there are ways to effectively employ data science to increase efficiencies and sales opportunities.
What Is Retail Data Science?
Data science is the art of taking a scientific approach, specifically by leveraging advanced computational methods to extract knowledge from all available data. This knowledge is then used to:
- Better serve an organization’s purpose
- Drive informed decision making
- Make more accurate predictions
- Enable better reactions to unexpected events
- Foster a permanent culture of learning and experimentation
Retail data science is specifically used to provide insights specifically useful for the retail industry. They’re used mainly to:
- Improve all aspects of the customer journey
- Personalize the customer experience
- Automate the buying and shipping processes to increase efficiencies
- Optimize operations to increase costs and revenue
For example, retail data science enables companies to react in real-time to disruptions caused by economic and weather changes.
- If a major port is hit with an ice storm and must unexpectedly shut down, do supply chain planners have enough data at their fingertips to create alternatives for an organization’s customers, or do they need a team of IT developers to help shift their focus?
- If rising transportation costs are cutting into revenue, is it better to find alternate delivery methods, get by on less stock, or raise costs?
These are the kinds of questions that retail data science helps businesses answer, leveraging accurate and up-to-the-minute information.
How Data Science is Transforming the Retail Industry
Processing data in real time enables all parts of the retail value chain to see the status of operations without delay and make better-informed decisions. The sheer amount of data to consider can be overwhelming, which is why combined data warehouses and data lakes — also known as lakehouses — use technologies to relevant data and meta-data to make better real-time decisions. Retail data science provides businesses with five general benefits:
- A better understanding of customers and their behaviors: The holy grail of retail is to be able to provide customers what they need when they need it. Thanks to data science, that goal is more attainable than ever:
- The use of sentiment analysis on customer reviews can create actionable insights to improve Net Promoter Scores (NPS).
- Formerly disparate data can be pulled together to support a truly 360-view of the customer, which can enrich each transaction and more accurately predict churn.
- Traditional demographics like age, gender, income level, and geography can be combined with newer insights on buying history, online presence, and digital behavior, allowing for more accurate (and timely) targeting.
- The use of sentiment analysis on customer reviews can create actionable insights to improve Net Promoter Scores (NPS).
- One-of-a-kind and completely seamless omnichannel experiences: Having access to more metrics to and the ability to target them makes it possible totailor customer interactions for a personalized — or even a hyper-personalized — experience. Additionally, connecting the physical store to the online world promotes a cohesive brand identity and provides customers with greater flexibility in how they shop and a more convenient experience.
- Optimized back-end operations, including inventory and supply chain management: One major lesson learned from the pandemic is how disruptions on the back-end can have long-lasting effects for retail customers. Real-time supplier forecasts improve safety stock estimates, while access to public data, such as current National Oceanic and Atmospheric Administration weather forecasts, enriches demand forecasting datasets to enable retailers to plan and prepare for what’s to come.
- Forecasting changes in demand: No, you can’t see the future, but you canmake a more educating guess about what may be coming. Data science can review historical data, market trends, behavioral data and more to inform predictive analytics to generate accurate demand forecasts.
- Optimization in pricing: A better understanding of how customers respond to different pricing levels in combination with outside trends, events and disruptions can help retailers better adjust product pricing. Retailers can be more confident in knowing how and when to competitively price products, or ways to promote and discount products that are less popular.
- Low Operating Cost: Lakehouses are cost efficient as all data is stored in cheap object storage like Azure Gen2 storage accounts. Additionally, all data remains under the firm’s ownership, there are no egress fees to get at your own data.
For specific examples of retail data science applications, here are 13 different use cases to consider.
13 Retail Data Science Use Cases You Need to Know
- Recommendation engines — Personalization is multifaceted, but at various points in the customer journey, organizations will have the opportunity to select content, products, and promotions to be presented to the customer. In these moments, retailers can take into consideration past feedback from customers in addition to larger trends to select the right items to present. Netflix has designed its personalization algorithm to update its recommendations based on a user’s personal viewing history in addition to what’s popular with other viewers who have similar tastes.
- Demand forecasting — This is the use of predictive analytics to estimate future customer demand to make smarter decisions about how to market, price, and stock products. generation of forecasts at a low level of temporal, geographic and product hierarchy granularity. Retail data science is able to hone in on “fine-grain” demand variables like seasonality, location, competition, and economic factors. forecasts have the potential to capture the patterns that influence demand closer to the level at which that demand must be met, especially during seasonal or promotional events. Walmart reviews social media data to stay on top of emerging products that can be quickly introduced into stores.
- Price optimization — Retailers can use product-level demand forecasts to calculate optimal prices, which will fluctuate dynamically depending upon real-time supply and distribution costs. While retailers have traditionally used price optimization to adjust the costs of select key value items, retail data science allows for a far more robust and dynamic adjustments to price based on demand, competition, economics and other factors. This approach not only provides customers with fair prices on the products they’re most interested in, it can allow retailers to more strategically set prices based on their priorities.
- Hyper-personalization — Retailers can take personalization a step further thanks to real-time AI-enabled processing of all customer data and historical sentiment. Whereas traditional personalization targets general personas, hyper-personalization can target individual persons when executing marketing campaigns, thanks to access to data like time and location of purchases, social media activity and even local weather. Sephora’s loyalty program is designed to provide specific “emotional perks” for customers, not just generic discounts and rewards.
- Trend forecasting — Retailers can use third-party data to keep tabs on leading trends, monitor customer behavior, and anticipate — and adapt to — changes in market demand. For an example of how retail data science supports trend forecasting, look to H&M: The company utilizes Big Data to predict trends three to eight months in advance and enhance merchandising by showcasing products popular within specific regions in its brick-and-mortar stores.
- Fraud prevention and detection — The longer the supply chain, the greater the risk for fraud or theft. Additional data points ensure that retailers can secure all points of their ecommerce chain from ordering and warehousing to shipping and delivery. Home Depot is employs prescriptive analytics to reduce shrinkage from waste, fraud, and abuse and to mitigate retail loss.
- Warranty analytics — Retailers now have the capability to connect warranty, customer satisfaction, and new product development datasets to surface the most cost-efficient improvements. Research from McKinsey shows that warranty analytics — specifically, AI and machine learning techniques — helped automotive companies lower expenses; increase their earnings before interest, taxes, depletion, and amortization by 1%–4%; and made buyers up to 18 times more likely to be repeat customers.
- Customer sentiment analysis — Thanks to advances in AI, it’s easier than ever to detect customer sentiments from text through natural language processing. As a result, retailers can develop actionable insights that can improve Net Promoter Scores by classifying sentiment in customer reviews and email responses to customer service interactions. A recent Nielsen survey highlighted shifting consumers expectations and priorities in the wake of the COVID-19 pandemic, and how companies should adjust to capitalize.
- Inventory and supply chain management — Internal data can detect inventory anomalies before they impact your customers. In the larger supply chain, a view of manufacturing and distribution capacity compared against anticipated demand and enriched with weather and social-economic disruption forecasts can help ensure that inventory stays stocked, and products are delivered in the most efficient manner. The fashion industry’s supply chain has seen a 10%–15% reduction in inventory costs thanks to data-driven efficiencies.
- Intelligent cross-selling and upselling — Showcasing products related to a customer’s purchase is a tried-and-true technique for retailers – whether a “buy the look” prompt from fashion brands like ASOS or Madewell or “pairs well with” furnishing suggestions from IKEA or Wayfair. Not everyone is primed for a cross-sell or an upsell, however, so retailers can now take social media feeds and historical sales into account when identifying those customers that are ready to buy more.
- Customer lifetime value prediction — In order to determine a customer’s lifetime value (CLV), retailers must compile and analyze historical sales data; take, for example, this example calculated via linear regression. Determining CLV enables retailers to carefully align customer acquisition costs and retention rates with total expected revenue, further optimizing marketing campaigns and better targeting repeat customers.
- Warehouse automation — Automation enables warehouse managers to expedite the picking and packing process, thereby accelerating delivery and reducing costs. Logistics suppliers like DHL and retailers like Walmart and Rofu Kinderland have already made major investments in autonomous mobile robot technology to staff their warehouses, a market which is expected to reach $51 billion by 2030.
- Entity resolution — Data isn’t always entered consistently or neatly, so entity resolution can be used to “clean” data and get an accurate and unified view. This technique is used to identify records within a data source (or sources) and connect them to a real-world entity. This can be used to:
- Resolve contract details and vendor names against financial transaction records to audit spend versus contract rates
- Merge product catalogs and price lists to reconcile pricing
- Unify customer data from across different silos to access a unified 360-degree view of the customer and eliminate duplicate records
How Hitachi Solutions Supports Retail Data Science
Microsoft Dynamics 365 allows for a retail customer engagement experience that’s consistent across physical and digital channels covering order management, CRM, ERP, digital marketing, customer care and more. As a global Microsoft solutions integrator, Hitachi Solutions delivers cutting-edge artificial intelligence for retail data science, including promotional demand forecasts, global sales forecasts, and natural language processing applications.
We offer low-cost entry points to help you jump start your data science practice with Advanced Analytics DataLab, a collaborative workspace that allows business users to take their real data and experience the modeling process. Our Microsoft D365 Tools suite helps to ensure that your operation is simplified and effective across new integrations, software updates, and code changes.
These are just a few specific examples of the tools and services we offer to help retailers maximize their Microsoft solutions – contact us to learn more.