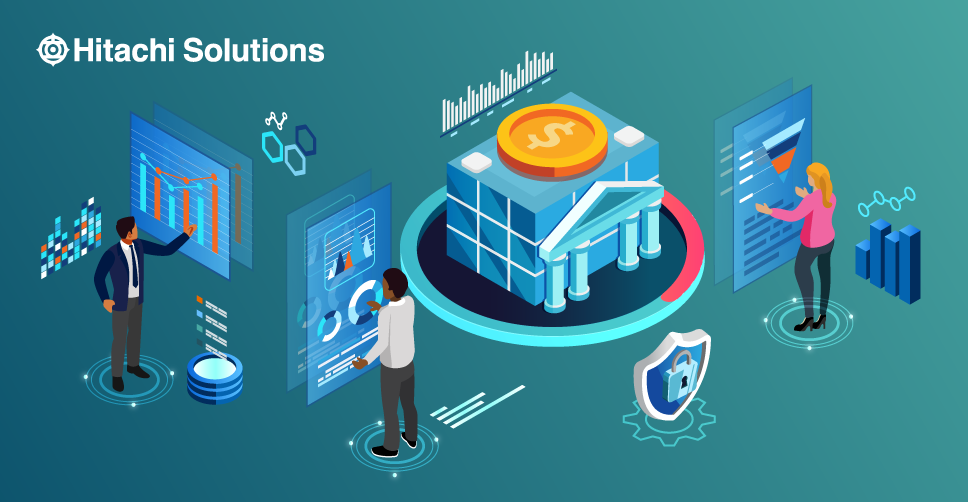
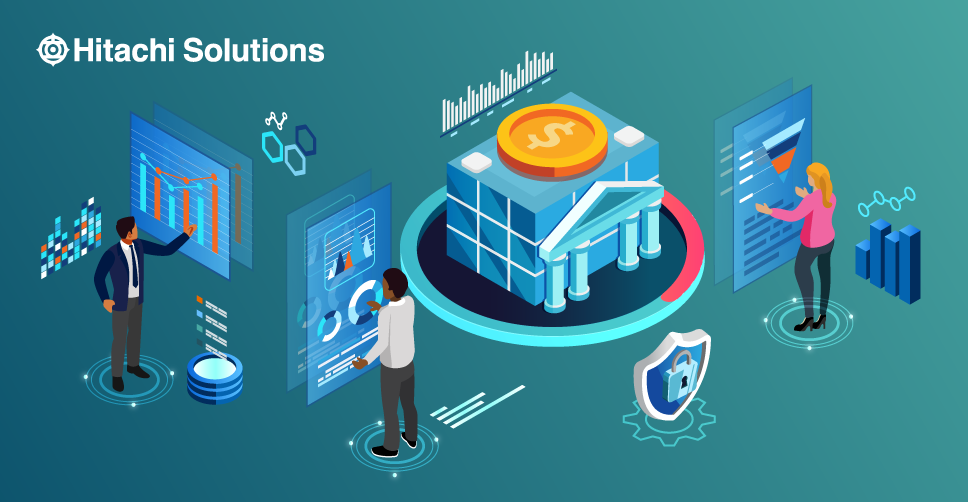
Digital transformation is no longer the future of the financial services sector — it’s the reality. Even prior to the COVID-19 pandemic, banks, credit unions, and other financial institutions were implementing innovative, digital solutions to address persistent challenges and to develop a more customer-centric approach to banking. That push has only accelerated over the past several years, due in no small part to the meteoric rise of so-called “disruptor banks” – mobile-first, app-based Fintech companies.
Many institutions have realized that outdated technology and changing customer expectations are some of their biggest industry challenges. According to Cornerstone Advisors’ What’s Going on in Banking 2022 study, three-quarters of banks and credit unions have already launched a digital transformation initiative. By the end of 2022, only 11% of banks and 4% of credit unions will not have launched some sort of digital transformation strategy. The financial intuitions that will remain competitive in this digital environment will be the ones that are able to value the customer experience over all else.
Of all the digital tools now available, banking analytics provides the means to enhance the customer experience, identify opportunities for revenue growth, and remain competitive in this increasingly volatile market. In this blog post, we’ll provide more background on and insight into the role of data analytics in banking, including ways in which financial institutions can benefit, common challenges, best practices, and more.
What is Banking Analytics?
Before we explain what banking analytics is, let’s take a moment to first discuss data analytics. According to Investopedia, data analytics is “the science of analyzing raw data in order to make conclusions about that information.” The raw data in question can be either structured or unstructured and come from either internal or external sources.
Organizations can leverage data analytics to do everything, from learning more about their customers and improving existing processes, to building predictive models and forecasting growth opportunities. Data analytics is a relatively broad term, one that encompasses many different forms of analysis, such as customer analytics, business analytics, predictive analytics, and so on. To that end, banking analytics simply refers to any application of data analytics in banking.
Data analytics has been integral to the way banks and other financial institutions do business for some time now; in fact, the financial services industry as a whole was one of the earliest adopters of analytics, having used it to monitor and anticipate sudden changes in the market. Nowadays, banks need to leverage banking analytics to derive granular insights from massive quantities of data — known as Big Data — and apply those strategic findings across all levels of business.
How Banks Can Benefit from Advanced Analytics in Banking
Banking analytics is an asset to any institution and provides a wide range of benefits, including:
A 360-degree view of the customer.
By applying advanced analytics to customer data — such as, say, which banking products they already have or who else is in their household — you can get an accurate picture of who your customers are, what motivates them, what matters to them, and so much more. You can even use sentiment analysis to capture how your customers feel about your institution.
This information enables you to pinpoint what it is your customers really need, rather than what you think they need. For example, you might mistakenly think that a customer is interested in a home loan, when that customer’s data — aggregated across multiple channels — indicates that they’re really looking to open an investment account. This level of insight can help you refine your sales and marketing efforts so that they deliver the right product or service to the right person at the right time, and therefore have a greater chance of success.
A superior omnichannel customer experience.
When we say, “deliver the right product or service to the right person at the right time,” we’re really talking about personalization in action. Personalization has really taken off as a trend in the banking industry, 72% of customers rate personalization as “highly important” in today’s financial landscape because it has the power to make customers feel seen, heard, and understood — all of which contribute to a better overall customer experience.
And customers aren’t the only ones who benefit: When done well, personalization can reduce customer churn and increase sales. According to research from Forrester, leaders in customer experience (CX) “grow revenue faster than CX laggards, drive higher brand preference, and retain more customers, who are more inclined to forgive stumbles made by brands with good CX.” According to one estimate, personalization can lead to annual revenue lifts of 10% for banks. None of this would be possible, of course, without customer analytics for banking.
Another way that banking analytics enhances the customer experience is by streamlining existing processes. Your customers are real people with busy lives; in order to earn and maintain their loyalty, you need to meet them in whichever channel they’re in and engage with them in a straightforward and seamless manner. It’s the best way to show that you value their time. For example, let’s say that you have an existing customer who wants to apply for a mortgage. Rather than ask them to wait while you track down paperwork — or worse, ask them to locate it themselves — you can save time and effort by storing all relevant data in a single, accessible location, which can be accessed at any time during any interaction, thereby expediting the loan origination process.
Data analytics solutions can point to these and other potential process improvements that optimize the customer experience and boost productivity within your workplace.
Stronger customer relationships.
By delivering the personalization that customers crave, demonstrating that you value their time and effort, and continually looking for ways to simplify or otherwise improve the customer experience, you can build stronger, longer-lasting customer relationships.
Customer attrition due to frustration and a lack of personalization is one of the biggest issues plaguing banks today. Forrester has found that, of banking customers who feel frustrated, only 21% planned to stay with the bank and to spend more with it, and a mere 13% will advocate for the bank. What’s more, a reported 57% of Gen X and Millennial customers would leave their financial institution to get proactive personalized services elsewhere.
Speaking of customer attrition, churn analytics are yet another way for financial institutions to leverage banking analytics to their advantage. Using churn analytics, you can identify weak spots within your organizations, come up with and test out theories as to why customers churn and determine which customers or customer segments are most likely to churn. Once you’ve determined where there’s room for improvement and which customers are at risk, you can take all of the knowledge you’ve gained from customer analytics and use it to develop strategies to ensure long-term customer retention.
Better risk management and mitigation.
There are a number of ways that banks can use data analytics to protect themselves from risk. For example, you could use customer analytics to segment customers into different groups based on their creditworthiness for the purposes of credit risk management. Not only does this enable you to narrow down your target audience for credit products, it also reduces your exposure to default risk because you can count on those customers to consistently make payments.
You can also use predictive analytics in banking to ward off potential fraud by analyzing customers’ behavioral patterns and flagging anomalous behavior. For example, let’s say that one of your customers typically logs into their account from a desktop with an IP address in Richmond, VA. One day, some attempts to log into that same account from a mobile device with an IP address in Albuquerque, NM. Since this deviates from the customer’s established behavioral pattern, your predictive analytics solution flags this as unusual activity and immediately notifies the customer so that they can take the appropriate action to protect their account.
Using advanced analytics for fraud detection and prevention not only protects the customer’s interests, but also your bank’s because it saves you from possible reputational damage or retaliatory action.
Lower operational costs.
Banks are under near-constant pressure to simultaneously reduce operational costs while increasing efficiency. In the past, financial institutions have attempted to thread this difficult needle by laying off staff, however, headcount reductions typically fail to get to the crux of the issue. In order to achieve this goal, banks need a long-term strategy rather than a quick fix.
That’s where banking analytics comes in. As we’ve already mentioned, you can use analytics to identify weak spots within your organization and shore them up accordingly. By applying that same logic, you can also use analytics uncover opportunities to jettison unnecessary expenses. You can even use a combination of predictive and prescriptive analytics to generate strategic recommendations on how to optimize existing processes — such as, for example, automating routine tasks — in order to drive efficiency and lower costs even further.
Growth opportunities.
As discussed, customer analytics makes it possible for banks to engage in more targeted sales and marketing — and the more attuned an offer is to a customer’s needs, the more likely your team is to close a deal. Banking analytics supports upselling and cross-selling strategies because it enables sales representatives to recommend complementary products and services that will deliver actual value to the customer rather than taking a wild shot in the dark. Data analytics in banking can even make it easier to determine which of your customers are the most profitable and create high-value customer segments that you can target in future sales and marketing initiatives.
Finally, data analytics has the power to help you identify new business models, especially in emerging markets. Insights derived from banking analytics — customer analytics, in particular — could have value to other B2B or B2C businesses, such as retailers and telecommunication companies. To that end, you could build out a new partner ecosystem, share relevant findings with partners, and come up with ways to combine your resources to create new business opportunities.
Obstacles to Implementing Data Analytics in Banking
As with adopting any technology, banking analytics comes with a slight learning curve. For those interested in implementing data analytics in banking, there are a few things you should be aware of.
First and foremost, strategy is everything. A well-defined strategy should be the foundation of any and every data analytics initiative. Without one, you can invest in all of the fancy tools and technology and still find yourself spinning your wheels. Any successful strategy starts with identifying which key goals and objectives you aim to achieve. Doing so not only provides you with a clear path forward, but also makes it easier to engage with key stakeholders. Every company-wide initiative requires executive buy-in, so being able to clearly articulate what your institution stands to gain from implementing banking analytics can help secure a “yes” from those in positions of power.
Failure to identify key goals and objectives has the downstream effect of making it difficult to determine which metrics and key performance indicators to track. Without a clear idea of what you’re working toward or how to measure success, it becomes more challenging for employees at all levels of your organization to get on board with banking analytics initiatives, which can impede organizational change management (OCM) and user adoption efforts. It also precludes you from leveraging advanced analytics in banking as effectively as you could or should: You might find yourself asking the wrong questions, thereby preventing algorithms from delivering actionable insights.
Once you have a strategy firmly in place, the next steps are to establish good data governance and to implement the right technology. Data governance ensures that all the big data in banking is collected in an ethical, compliant, and accountable manner. It sets important guidelines for where data is stored, how it’s accessed and how it’s used — all of which ensure that your employees are supported and that your data processes are sustainable.
It’s then necessary to replace outdated legacy systems that keep data siloed across different lines of business — even from one team member to the next. These silos require both customers and customer-facing agents to put in more effort than is necessary, which slows down processes and negatively affects the customer experience. Therefore, it’s imperative that banks and other financial institutions invest in advanced analytics solutions that enable them to bridge islands of data to resolve issues and deliver financial advice in a timelier fashion.
12 Tips for Developing a Successful Banking Analytics Strategy
When developing a banking analytics strategy, there are a few best practices you should follow in order to set yourself up for success:
- Start small and grow your strategy over time. There’s no sense in trying to get everything done all at once — attempting to do so is impractical, expensive, and unlikely to deliver the outcome you’re looking for. It’s best to start small, figure out what works and what doesn’t, and go from there. Best of all, you can use small wins to finance future projects, allowing for the greatest ROI.
- Learn by trial and error. Speaking of figuring out what works and what doesn’t, implementing a banking analytics strategy should be an iterative process; every individual project should be viewed as an opportunity to learn something meaningful about how your different lines of business and, indeed, your institution as a whole work.
- Adopt what works and eliminate what doesn’t. We’re really just driving home the point here. That said, it’s important to add that you shouldn’t hold on to any processes, policies, tools, and so on that no longer serve your interests, no matter how accustomed your employees may be to them. Sometimes it’s best to let go of what’s familiar in favor of what’s effective.
- Build a data ecosystem using internal and external sources. If your bank only relies on internal data, you’re only getting half of the story. External data can provide valuable context to internal findings, so it’s important that you fold in external data sources whenever and however possible.
- Gather customer insights across multiple data sets. Sometimes the most valuable information comes from the most surprising places, so look for insights into all possible data sets. The best way to develop a full 360-degree view of your customers by investing in a customer data platform. This will collect customer data across every point of contact and allow that knowledge to be shared across the entire institution.
- Ask the right questions. In order to do that, you first need to know what the right questions are. This is where knowing exactly what it is you’re looking for and what you hope to achieve really come in handy. In order to avoid wasting precious resources, first figure out what it is you hope to achieve, and what questions will help you glean the most information.
- Invest in OCM. Organization-wide adoption of any digital transformation doesn’t just happen by chance — it requires hard work, determination, and an effective OCM strategy. To properly implement and manage banking analytics, you’ll need to follow a structured process:
- Start by analyzing the company landscape to establish your long-term visions and more immediate goals and metrics.
- Review and measure the technology and training investments that you will need to make.
- Communicate the changes to your employees, how they will be impacted and how they and the institution will benefit.
- Invest in training to help employees adjust to the new systems, processes, and protocols.
- Measure outcomes to ensure that all KPIs and ROI goals are on-track.
- Set up a feedback loop so that employees can share what’s going well and where they struggle; implement a support structure so that they have someone or somewhere to turn to should they encounter issues.
- Finally, revisit your OCM strategy at a later date to determine whether it was successful and how to further support banking analytics adoption going forward.
- Look for systems and solutions that are easy and intuitive to use. The fact of the matter is that people are unlikely to adopt and use systems that are overly complex, clunky, and confusing. When evaluating different banking analytics solutions, look for one that uses aesthetically pleasing and intuitive visualizations and dashboards so that data-driven insights are easy to access, understand, and leverage. You can ensure success by training your personnel on business intelligence (BI), reporting, and data visualization products and services.
- Lean on executives to role model new behaviors. Executive buy-in isn’t just necessary to get banking analytics off the ground, it’s essential for ensuring that employees at all levels of business get on board with new systems and strategies. During the planning stage of your institution’s banking analytics initiative, make it clear to executives — and those in managerial positions — that you’re counting on them to act as role models to lower-level employees in order to ensure adoption.
- Ensure alignment with performance metrics, KPIs, and governance. Beyond the executive buy-in, the entire institution will need to follow through on its established strategies and evaluate effectiveness on a regular basis. Have strategies in place to measure outcomes, determine which metrics are relevant, and make any changes or adjustments needed. Keeping your systems synchronized through good governance and master data management will keep your data updated, secure, and accessible.
- Automate as much as you can. By automating the fulfillment of low-level service requests, such as adding someone to an account or adding a new credit card, you can save agents valuable time, thereby enabling them to focus on more high-level, high-value requests.
- Assemble a winning team. When implementing a banking analytics strategy, it’s important to have a team of professionals that not only have data science expertise, but also relevant industry experience to support you. Hitachi Solutions is that team: For over a decade, we’ve been working with institutions in the financial services sector to overcome top business challenges, deliver personalized customer experiences, defend against cybercrime, ensure regulatory compliance, and remain competitive, all using data analytics.
Create a Customer-Centric Approach to Banking with Hitachi Solutions
Here at Hitachi Solutions, we specialize in helping organizations across all industries — including the financial services sector — craft exceptional customer experiences by leveraging the power of data analytics. From defining a winning banking analytics strategy to identifying the appropriate tools and technologies to implement, Hitachi Solutions is here to assist you at every step of your journey to becoming the bank of the future.
Contact us today to find out how to take your banking customer experience to the next level.
Frequently Asked Questions
Q: What is banking analytics?
A: Banking analytics refers to the application of data analytics — that is, the use of various tools and technologies to collect, process, and analyze raw data — within the banking industry. Examples of banking analytics include customer segmentation, credit risk management, and fraud detection.
Q: Why is banking analytics important?
A: Banking analytics is important because it enables banks, credit unions, and other financial institutions to turn the massive quantities of data they create or consume into valuable, actionable insights. Data analytics in banking is a powerful tool for improving performance, optimizing the customer experience, and growing revenue, amongst other things.
Q: What is customer analytics in banking?
A: Customer analytics is a subset of banking analytics, which banks can use to gain a 360-degree degree view of their customers. This provides banks with a better understanding of what it is their customers want and need, so they can offer more targeted product and service recommendations (which improves upselling and cross-selling efforts) and personalize every customer interaction. By leveraging customer analytics in banking, it’s possible to build stronger customer relationships and improve the overall customer experience.
Q: What is predictive analytics in banking?
A: Predictive analytics refers to a form of data analytics where organizations use advanced technology, such as artificial intelligence, data mining, and machine learning, to develop models that “predict” events that are likely to occur. In banking, predictive analytics is often used to help customers manage their finances, prevent fraud and other forms of identity theft, and mitigate risk.
Q: How can banks benefits from using data analytics?
A: There are seemingly endless ways to apply data analytics in banking, boosting customer retention, including optimizing existing processes, reducing operational costs, enhancing productivity, and so on. When it comes to banking analytics, financial institutions are only limited by their imagination and the technical infrastructure underpinning their analytics strategy.
Q: How can banking analytics improve the customer experience?
A: There are any number of ways for banks to leverage analytics to enhance the customer experience, from creating more targeted marketing campaigns to providing personalized product and service recommendations. Ultimately, the purpose of banking analytics — or, more specifically, customer analytics in banking — is to gain insight into each individual customer, so you can tailor the entire banking experience to cater to their unique wants, needs, interests, and motivations.
Q: How does cloud technology support data analytics in banking?
A: Although it’s possible to use legacy systems for banking analytics, you can’t tap into its full potential unless you run your analytics strategy on a modern cloud infrastructure.