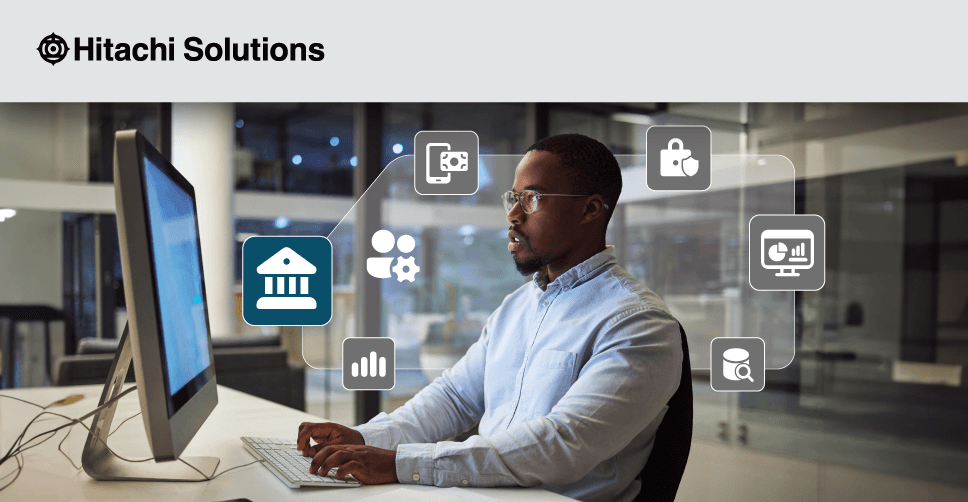
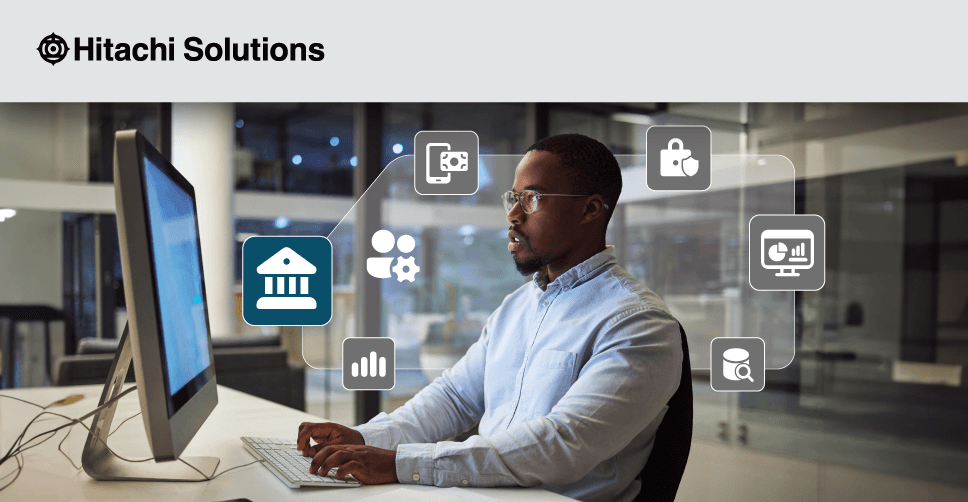
Innovate with AI and Cloud Scale Databases in Every App
Join our expert discussion to learn about cloud use cases and the innovative potential for AI in your business.
Watch the On-Demand WebinarBanking customers generate an astronomical amount of data every day through hundreds of thousands — if not millions — of individual transactions. This data falls under the umbrella of big data, which is defined as “large, diverse sets of information that grow at ever-increasing rates.” To give you an idea of how much information this is, we generate 2.5 quintillion bytes of data every day! This data holds untapped potential for banks and other financial institutions that want to better understand their customer base, product performance, and market trends.
But where, exactly, does all this data come from? The technology behind smartphones, tablets, and the Internet of Things (IoT) has made it easier than ever for customers to use online resources to communicate with companies, research products, purchase items, and even perform banking tasks. These activities generate first party data, which can then be combined with select third party data such as market and demographic trends to inform banking analytics.
When properly utilized across all departments, data analytics in banking can help institutions develop customer profiles that enable them to anticipate behaviors, identify cross-selling opportunities, deliver more proactive and empathetic service, and better manage risk. The end result? Renewed trust between customers and their banking institutions and an elevated customer experience that inspires long-term loyalty.
The 4 Types of Data in Modern Banking Analytics
With 2.5 quintillion bytes of data generated every day, not all of it can fit within a single category. There are three ways to classify big data:
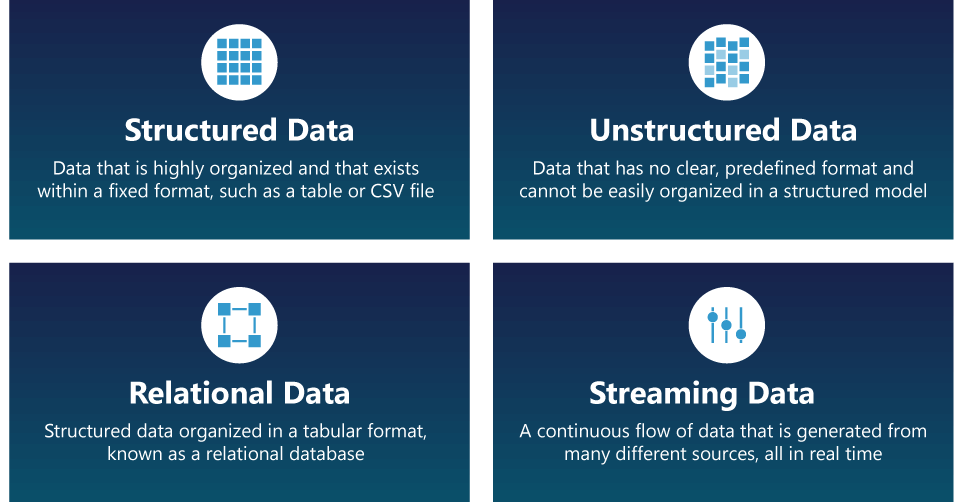
- Structured Data: Structured data refers to data that is highly organized and that exists within a fixed format, such as a table or CSV file, with each element having a specific and predefined meaning. Structured data is typically easier to query and analyze than unstructured data.
- Unstructured Data: Unstructured data refers to data that has no clear, predefined format and, therefore, cannot be easily organized into a structured model for querying and analysis. Unstructured data can take many forms, such as text, images, audio files, and videos.
- Relational Data: Relational data refers to structured data organized in a tabular format, known as a relational database. Each row within a relational database holds a record with a unique identifier, or key, and each column holds the attributes of the data. This format enables organizations to not only organize data, but to identify relationships between tables, which allows for flexible querying and analysis.
- Streaming Data: Streaming data refers to a continuous flow of data that is generated from many different sources. In the banking industry, these sources may include credit card transactions, stock market data, customer service interactions, and ATM transactions. Streaming data is often processed and analyzed in real time.
The incredible volume of data available at our fingertips requires advanced processing techniques in order to be translated into valuable, actionable information. Using the proper business tools is the most efficient way to filter through all types of big data.
The Role of Banking Analytics
The banking industry is a prime example of how technology has revolutionized the customer experience. Gone are the days when customers had to stand in line on a Saturday morning just to deposit their paycheck. Customers can now use their mobile phone to check their account balances, deposit checks, pay bills, and transfer money — there’s no need for them to even leave the house.
These self-service features are fantastic for customers, but they are one of the main reasons why traditional banks are struggling to compete with similar businesses and online-only financial institutions. Since customer activity now occurs mostly online, certain in-person services that brick-and-mortar banks have been known to provide are no longer relevant to customer needs.
This is where adopting advanced analytics strategies and tools becomes so important to the banking industry. Using both personal and transactional information, banks can establish a 360-degree view of their customers in order to:
- Track customer spending patterns
- Segment customers based on their profiles
- Implement risk management processes
- Personalize product offerings
- Proactively address customer needs
- Incorporate retention strategies
- Collect, analyze, and respond to customer feedback
Using analytics-driven strategies and tools, banks are able to unlock the potential of big data to great effect: across all U.S. industries, companies that can move into the top quartile in personalization performance are projected to collectively generate more than $1 trillion in value.
5 Key Benefits of Modern Data Analytics in Banking
Let’s take a closer look at some of the benefits banking analytics has to offer:
Gain a Complete View of Customers Through Profiling
Customer segmentation has become commonplace in the banking industry because it enables institutions to profile customers by analyzing a wide range of data points, helping them better understand customer preferences, behaviors, and needs.
To support segmentation, banks need to be able to collect massive volumes of customer data from a variety of internal and external sources. They also need to be able to store virtually unlimited volumes of data to analyze and mine it for insights. Customer data platforms not only offer data gathering and storage capabilities, but enable banks to develop hyper-personalized customer profiles that account for a wide variety of factors, including:
- The customer’s demographic
- Their number of active accounts
- Which financial products they’ve purchased
- Which offers they’ve declined in the past
- Which products they’re likely to purchase in the future
- Major recent and upcoming life events
- Their relationship to other customers
- Attitude toward their bank and the financial services industry as a whole
- Behavioral patterns
- Service preferences
- And more
Armed with this information, banks can engage in the following:
- Demographic Analysis
By analyzing demographic data, including age, gender, income, occupation, and location, banks can better understand the needs and preferences of different customer groups and tailor their products and services accordingly.
- Sentiment Analysis
Sentiment analysis is a powerful way for banks to understand how customers perceive their products, services, and even organization as a whole. By applying data analytics to social media data, customer reviews, and other online services, banks can not only determine social sentiment, but also flag areas of improvement and adjust their strategies accordingly.
- Behavioral Analysis
By analyzing transaction history, banks can recognize patterns in spending habits, product usage, and preferred channels of interaction (that is, online, mobile, or in-person). This information enables banks to create targeted marketing campaigns and offer personalized services to meet the specific needs of each customer segment.
- Social Network Analysis
By examining customer connections on social media platforms, banks can identify influencers, brand advocates, and potential new customers. Social network analysis helps banks understand customer interests and preferences, enabling them to create targeted marketing campaigns and personalized services.
- Credit Scoring
Data analytics is an excellent way for banks to assess the creditworthiness of potential borrowers. Banks can apply analytics to customer data such as income, credit history, and current debt levels to generate credits, which help them determine the risk associated with lending to a particular individual.
- Predictive Analytics
By applying banking analytics to historical customer data and applying machine learning algorithms, financial institutions can predict future customer behavior and preferences. This information enables them to anticipate customer needs and offer proactive solutions, such as recommending new products or services.
- Customer Lifetime Value Analysis
Customer lifetime value (CLV) refers to the anticipated total net profit that a customer will generate for a bank over the course of their relationship. Financial institutions can apply banking analytics to a customer’s transaction history, demographic data, and interactions to estimate their CLV. Banks can then leverage the results of this analysis to optimize their marketing efforts and allocate resources effectively.
- Churn Prediction
Nearly half of all Americans — 45% — considered changing banks in 2021, citing a wide variety of reasons. To avoid loss of business, banks need a way to identify customers who are likely to leave for a competitor and proactively respond. By analyzing factors such as transaction frequency, account balances, and customer interactions, banks can implement strategies to retain high-value customers and reduce churn.
- Personalized Marketing
Using customer profiles, banks can create personalized marketing campaigns that resonate with specific customer segments. Personalized marketing improves customer engagement and conversion rates by delivering relevant and timely messages.
Tailor the Customer Experience to Each Individual
Across all industries, nearly three-quarters of customers rate personalization as “highly important” in today’s financial services landscape. The stakes are high, as a reported 57% of Gen X and Millennial customers would leave their financial institution if they would receive proactive personalized services elsewhere.
For all its talk of relationship banking, the financial services industry isn’t exactly known for its high level of personalized service. Almost half of surveyed banks and credit unions surveyed by the Financial Brand in 2021 admitted that their customer relationship management (CRM) system wasn’t providing helpful analytics or recommendations. For those banks and credit unions that hope to not only survive, but thrive, a banking analytics-oriented shift in perspective and tailor-made customer experience are absolute necessities.
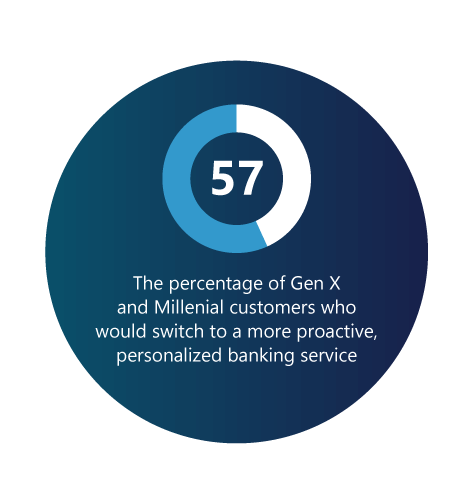
Understand How Your Customers Buy
Almost all big data in banking is generated by customers, either through interactions with sales teams and service representatives or through transactions. Both forms of customer data have immense value, as transactional data offers banks a clear view of their customers’ spending habits and, over time, larger behavioral patterns.
However, data by itself won’t necessarily provide insights into a customer’s preferences and behavior — unless it is collected into a centralized customer data platform. A CDP gathers all available first-party customer data from a range of sources, including interactions and transactions from CRM tools, websites, mobile apps, and branch locations. All that data is then placed into a single storage repository and can be supplemented with data collected from third-party sources to better inform banking analytics.
Identify Opportunities for Upselling and Cross-selling
According to research from HubSpot, 72% of salespeople who upsell and 74% who cross-sell say that it drives up to 30% of their revenue. It’s clear that upselling and cross-selling — that is, identifying opportunities to offer premium or upgraded versions of products and complementary products — presents easy opportunities for banks to increase their profit share.
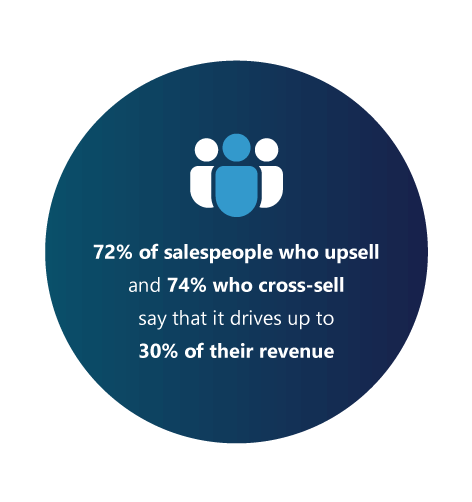
Institutions can take advantage of these opportunities by integrating behavioral and transactional data into customer profiles and making those profiles accessible to employees online, in-branch, and at other customer touchpoints.
Reduce the Risk of Fraudulent Behavior
Identity fraud is one of the fastest-growing forms of fraud, with the Federal Trade Commission stating that 1.4 million cases have been reported in the U.S. so far in 2023. Monitoring customer spending patterns and identifying unusual behavior is one way in which financial institutions can leverage banking analytics to prevent fraud and make customers feel more secure.
Banking Analytics in Action: A (Fictionalized) Customer Story
To illustrate just how financial institutions can take advantage of modern data analytics in banking, let’s follow the journey of a fictional customer, Avery, who recently opened a primary checking account with America One, a fictional bank.
After years of dissatisfaction with their previous bank, Avery recently made the switch to America One at the recommendation of a few of their friends. Avery’s excited to be with America One because they’ve heard great things about its personalized customer service — and America One is excited to have Avery, too.
Now that Avery’s officially a customer, America One’s team is ready to use big data and banking analytics to ensure that they have the best experience possible.
According to America One’s customer profile of Avery, they are in their late 30s, which means they’re a member of Generation X. Avery is college-educated, lives just outside a major metropolitan area, and has been married to their partner — who is also an America One customer — for the past four years.
When Avery joined America One, they were earning a median salary, but a recent promotion has pushed them into a higher income bracket. At present, Avery has two accounts — a primary checking account and a high-interest savings account — and a credit card with America One. As a homeowner, Avery also has a home mortgage with a different bank.
Avery’s a big fan of online banking; they check their accounts at least once a day through America One’s mobile application. They have only submitted two service requests to date, both of which were resolved within 24 hours.
By looking at Avery’s customer profile and service history, an American One employee can see that Avery prefers to do most of their banking online using the bank’s mobile app. Based on this data, and data from other customers with similar preferences, America One’s executive leadership team decides to add an AI-enabled virtual agent functionality to its apps so that customers can submit service requests and resolve issues entirely online.
Since the virtual agent uses AI technology to analyze Avery’s data and identify behavioral patterns, it can accommodate their preferences and provide personalized responses without ever sacrificing the quality of service. Should Avery’s request exceed the virtual agent’s capabilities, or should they decide that they’d like to talk to a human, it will automatically escalate their request to a live service representative.
America One already knows what Avery’s monthly paycheck is, that they like to pay their bills early, and that they put an average of $500 into a high-interest savings account per paycheck. This information provides a solid foundation for who Avery is as a person, such as that they’re a relatively high earner with disposable income, have a high credit score, are responsible about their monthly payments, and value saving money for the future.
Seeing that their savings account currently holds a balance of over $10,000, America One offers Avery a high initial rate CD during their next login and suggests that they talk to an in-house financial advisor to learn how much they could earn in higher interest by putting that money to work.
One day, while reviewing Avery’s transaction history, an America One employee notices that Avery recently purchased plane tickets for them and their partner to a few different cities across Europe and South America, as well as booked hotels for each location. Based on this information, the employee (as it just so happens, correctly) assumes that Avery is passionate about travel.
The employee then pulls up Avery’s customer profile, which shows that they already have one credit card with America One, but that their credit utilization is slightly low. Seeing an upselling opportunity, the employee targets Avery with a marketing campaign for America One’s travel rewards card, which they can use to earn airline miles while increasing their credit utilization and improving their credit score in the process.
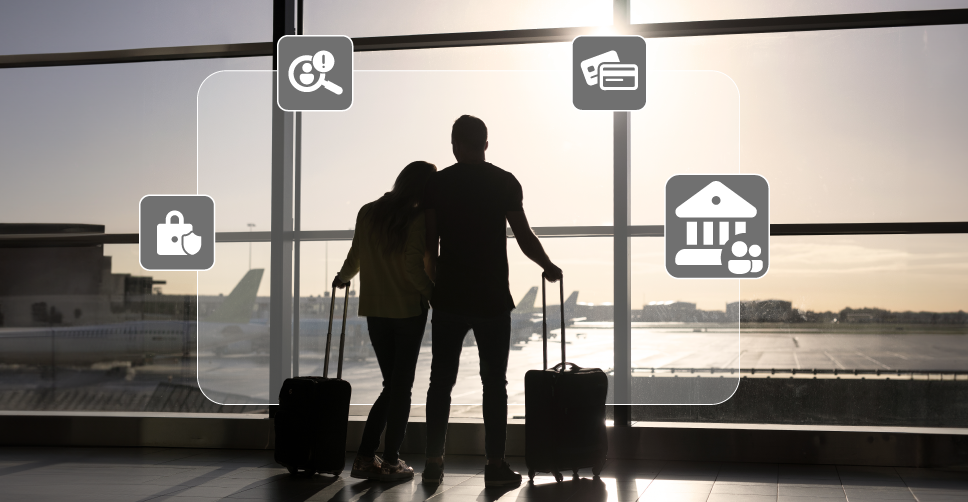
Prior to embarking on a trip to Barcelona, Avery notifies their bank that they’ll be traveling out of the country so that it won’t put a freeze on their account while they’re abroad. However, while Avery is on the trip, an America One employee notices that someone attempts to withdraw money from their account from an ATM in Houston, TX — over 1,000 miles away from Avery’s hometown in Chicago, IL, and over 5,000 miles from their current location in Barcelona.
Suspecting fraudulent activity, the employee pulls Avery’s phone number from their customer profile and contacts them directly to notify them. After confirming that it is, indeed, fraudulent activity, the employee denies the ATM request, thereby keeping Avery’s account safe.
5 Things to Watch for When Implementing Banking Analytics
Implementing a modern data analytics strategy in banking is in the best interest of any financial institution, but it isn’t without its challenges. There are a few things banks and credit unions should be aware of before they proceed.
- Legacy systems lack the infrastructure to accommodate banking analytics. The sheer volume of big data puts a considerable strain on legacy systems, and many legacy systems lack the advanced analytics in banking to make sense of it in the first place. Banks are therefore advised to upgrade their existing systems before implementing a modern data analytics strategy.
- Data quality management needs to be a top priority. Even if a bank upgrades its system, dirty data — data that is inaccurate, inconsistent, incomplete, duplicate, or outdated — can skew results. Prior to the digital age, most data was entered manually, thereby introducing the risk of human error. Banks should carefully review and consolidate their existing data before they enter it into a new system to identify and eliminate instances of dirty data and, in the future, authenticate data input sources to reduce new instances of dirty data.
- Customers are concerned about the state of data privacy. With multiple security breaches making the news — most recently, a hacker gained access to 100 million Capital One accounts — bank and credit union customers are on high alert over the security of their sensitive data. Banks that hope to capitalize on big data also need to implement robust security measures, such as two-factor customer authentication, data encryption, and real-time and permanent masking, to allay customers’ fears.
- Consolidation is crucial after an acquisition. Any time an acquisition occurs, new databases are added to a bank’s data estate. In order to be analyzed and put to effective use, the data spread out across each of these disparate systems needs to be consolidated in a central repository. By consolidating data in the immediate aftermath of an acquisition, financial institutions can more easily identify and eliminate dirty data and prevent employees from having to comb through multiple systems to locate relevant customer and product data.
- Financial institutions are subject to more rules and regulations than ever before. From FINRA to FinCEN to the much-talked-about General Data Protection Regulation (GDPR), banks are under mounting pressure to remain compliant with an ever-growing list of data-related regulations and regulatory agencies. In order to ensure compliance, banks and credit unions need to go above and beyond when it comes to security and risk management.
10 Use Cases for Banking Analytics
Modern data analytics has transformed the banking industry, enabling institutions to make more informed decisions, continuously improve the customer experience, and enhance their services. Here are just a few examples of how you might leverage modern data analytics in banking:
- Customer Segmentation
Banks can use data analytics to segment their customers based on demographic information, transaction history, products owned, and behavioral patterns. By segmenting customers and developing detailed customer profiles, banks can create targeted marketing campaigns, tailor their product offerings, and deliver highly personalized services.
- Fraud Detection
Fraud is a serious concern for banks everywhere, with one study showing that the average volume of monthly fraud attacks for banks increased from 1,977 in 2020 to 2,320 in 2021 — a 17.3% increase. Banking analytics can help financial institutions recognize unusual patterns and anomalies in transactions, which could point to potential fraud. Additionally, by analyzing customers’ historical transaction data, banks can build predictive models to identify and prevent fraudulent activities in real-time.
- Risk Management
Financial institutions can leverage banking analytics to assess and manage various risks, including credit risk, market risk, and operational risk. By analyzing historical data, banks can build predictive models to identify potential risks and take proactive measures to mitigate them.
- Virtual Agents & Assistants
Banks’ contact centers are often flooded with low-level questions and requests that could be easily addressed through self-service. The more time service representatives spend responding to these requests, the less time they’re able to devote to more complex issues, which leads to poor resource allocation and higher costs for banks.
But for every problem, data analytics in banking has a solution: Institutions can use natural language processing (NLP) and machine learning algorithms to develop virtual agents and assistants, which can help customers with account inquiries, transactions, and general support, thereby reducing the load on live agents.
- Algorithmic Trading
Investment banks can leverage advanced analytics to support algorithmic trading. Using machine learning algorithms, these banks can analyze market data, identify trading opportunities, and automatically execute trades — all with minimal human intervention.
- Network Analysis
Financial institutions can use banking analytics to study the relationships between various entities, including individuals, businesses, and organizations. This analysis enables banks to identify potential risks, detect fraud, and uncover new business opportunities.
- Enhanced Customer Experiences
Data analytics in banking will continue to drive improvements in customer experience, enabling banks and credit unions to offer more personalized services, seamless omnichannel experiences, and proactive solutions to meet customer needs.
- Workplace Development & Upskilling
As data analytics becomes more central to banking operations, institutions will need to invest in workforce development and upskilling programs to ensure employees have the necessary skills to leverage data analytics effectively.
Advanced analytics in banking will also give institutions the opportunity to monitor performance metrics, assess employee feedback and company culture, and gauge overall employee satisfaction.
Finally, banks will be able to empower their employees through the use of analytics, arming them with the information they need to deliver gratifying experiences to customers.
- Predictive Maintenance
Banking analytics has the ability to help banks keep essential hardware, such as ATMs and servers, online through predictive maintenance. By collecting and analyzing operational, physical, and performance data from equipment and applying machine learning algorithms to that data, banks can identify patterns that correlate to device failure. These patterns then enable banks to develop predictive models to forecast failure probability and trigger proactive maintenance when risk is high.
Predictive maintenance powered by banking analytics can help banks save on costs, improve operational efficiency, enhance customer satisfaction due to reduced outages, and extend the lifespan of hardware.
- Regulatory Compliance
Advanced analytics in banking can help institutions adhere to anti-money laundering and know your customer rules, as well as other important regulations, by enabling them to monitor transactions in real time, detect unusual patterns, and flag potential risks. Analytics also enables banks to build comprehensive risk profiles of customers and automate suspicious activity reporting and sanction screening. Banking analytics can even help institutions track changes in regulatory requirements, thereby reducing their compliance costs, avoiding penalties, and mitigating risk to the business.
The Future of Banking Analytics
Financial institutions are finding new ways to harness the power of modern data analytics in banking every day — a journey of discovery driven by technological innovation. Looking to the future, here’s what we expect to see from modern data analytics in banking:
- Increased Adoption of Artificial Intelligence & Machine Learning
Artificial intelligence (AI) and machine learning will play an even more significant role in banking as they become more sophisticated and are integrated into various banking functions. These technologies will enable banks to automate tasks, make better predictions, and enhance decision-making processes.
- Advanced Analytics in Banking Techniques
Banks will increasingly use advanced analytics techniques such as deep learning, graph analytics, and NLP to gain deeper insights into customer behavior, preferences, and needs. These techniques will help banks improve their products and services and create more personalized customer experiences.
- Real-time Analytics
The demand for real-time banking analytics will continue to grow, enabling institutions to respond quickly to changing market conditions, customer needs, and preferences. Real-time analytics will enable banks to create timely and relevant marketing campaigns, enhance customer service, and make more informed decisions.
- Enhanced Data Security and Privacy
As data becomes more valuable, banks will need to focus on enhancing data security and privacy. This may involve adopting advanced encryption techniques, implementing stricter access controls, and ensuring compliance with data protection regulations such as GDPR and the California Consumer Privacy Act.
- Integration of External Data Sources
Banks will increasingly integrate external data sources, such as social media, web browsers and IoT devices, to create more comprehensive customer profiles. By applying banking analytics to data gathered from these sources, institutions will be able to offer more personalized products and services, as well as identify new business opportunities.
- Growth of Open Banking & APIs
Open banking initiatives and the increased use of APIs will enable banks to share data and collaborate with third-party providers, such as fintechs and insurtechs. This shift will lead banks to develop new products and services and improve customer experiences, and will increase competition in the banking sector.
- Cloud-based Data Analytics
The adoption of cloud-based banking analytics platforms will continue to grow, providing institutions with scalable, cost-effective, and flexible solutions for their data analytics needs. Cloud-based platforms will enable banks to access and process large volumes of data more efficiently and securely.
- Ethical AI & Responsible Data Usage
As AI — including large language learning models, which aim to replicate human speech — and data analytics become more pervasive, banks will need to consider the ethical implications of their use. This may involve developing guidelines and best practices for responsible data usage, ensuring transparency in AI decision-making processes, and addressing potential biases in data and algorithms.
Embrace Modern Data Analytics in Banking with Hitachi Solutions
One of the biggest challenges facing the banking industry is that many legacy systems aren’t equipped to handle big data or modern analytics. And although the concept of big data in banking has been around for several years now, many institutions have yet to build an infrastructure capable of handling the high volume of information that comes with it.
Are you ready to rethink your infrastructure and discover the true potential of big data in banking? Hitachi Solutions is the perfect partner to help you do it.
Our Empower data platform is designed to help businesses across all industries consolidate massive quantities of internal and external data, manage it in a cost-effective way, and extract valuable insights using business intelligence and advanced analytics.
See for yourself how you can modernize banking analytics with Empower — sign up for our Empower for Financial Services trial, or contact the Hitachi Solutions team today.